17 minute read · August 22, 2024
The Value of Self-Service Data and Dremio’s Self-Service Capabilities

· Head of DevRel, Dremio
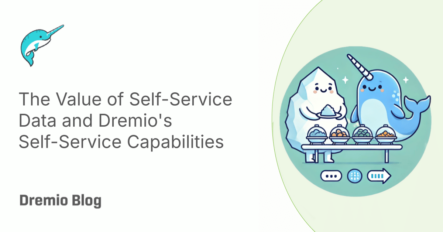
The ability to access and analyze data efficiently can be a game-changer for businesses. However, the traditional data pipeline—where data engineers meticulously ingest, model, and deliver data to various stakeholders—often becomes a bottleneck. Data consumers, from analysts to business users and data scientists, frequently find themselves waiting for the right data, the right model, or the right dashboard, leading to delays and iterative cycles that can stretch into weeks. This status quo not only slows down time-to-insight but also increases costs and frustration. Enter self-service data capabilities—a paradigm shift that empowers data consumers to access, explore, and utilize data closer to the source, with the autonomy to fulfill many of their needs directly. Let's explore the value of self-service data, the critical functionalities needed to make it work, and how Dremio’s robust platform is uniquely positioned to deliver on this promise.
The Challenges of Traditional Data Workflows
The current state of data workflows in many organizations is heavily dependent on a sequential process that involves multiple stakeholders, each playing a crucial role in moving data from raw sources to actionable insights. However, this traditional approach is fraught with challenges that can slow down the entire process and create inefficiencies.
Data Engineers as Gatekeepers
In most organizations, data engineers are responsible for ingesting data from various source systems into a data lake then into a centralized data warehouse. They are tasked with cleaning, transforming, and modeling this data to create structured datasets that serve the needs of different business lines. These datasets are often packaged as data marts or specific data products, designed to answer predefined questions or support specific business functions.
However, this process is time-consuming. Data engineers must balance the needs of multiple stakeholders, each with their own requirements and timelines. This often leads to bottlenecks, where data consumers—whether analysts, data scientists, or business users—are left waiting for the data they need. The delays are further exacerbated when requirements change or when new data sources need to be integrated, necessitating additional rounds of data engineering work.
Data Analysts and the Burden of Extracts
Once the data engineers have delivered the data marts, analysts take over. They query these datasets to create reports, visualizations, and dashboards that are used by business users to make informed decisions. However, this often involves creating extracts—copies of the data—that are pulled into various BI tools. These extracts can become stale quickly, requiring frequent updates and maintenance, which adds to the overall time and effort required to deliver insights.
Moreover, because analysts are often working with pre-structured data, they may need to go back to the data engineers if they require additional data points or if their analysis uncovers new questions that the existing data marts cannot answer. This iterative process can take weeks, delaying critical business decisions and increasing the likelihood of miscommunication or misunderstandings.
Business Users and the Pain of Iteration
For business users, the reliance on data engineers and analysts creates a disconnect between the questions they want to ask and the data they can access. By the time a dashboard or report is delivered, the business landscape may have changed, or the initial requirements may have evolved. This leads to a cycle of revisions, where business users request changes, analysts rework their queries, and data engineers may need to adjust pipelines—all of which adds to the cost and time required to deliver actionable insights.
Data Scientists and the Feature Engineering Dilemma
Data scientists face similar challenges. While they have the expertise to build complex models, they often rely on data engineers to provide the datasets they need. When a model requires a new feature—perhaps a specific data point or a new data source—they must wait for the data pipelines to be updated. This not only slows down the development of the model but also increases the risk of missed opportunities, as data scientists may be unable to iterate quickly enough to keep up with the demands of the business.
The High Cost of Delays and Revisions
The traditional data workflow, with its inherent delays and iterative cycles, has significant cost implications. Each revision, whether it’s a change to a data pipeline, a new extract, or a dashboard update, consumes compute resources, storage, and engineering time. These costs are magnified by the increased time-to-insight, which can lead to missed opportunities and slower decision-making.
To address these challenges, organizations need to rethink how they provide data access to their users. This is where self-service data capabilities come into play, offering a way to empower data consumers to fulfill many of their needs directly, without relying on the traditional data pipeline.
The Power of Self-Service Data
Self-service data is not about bypassing the expertise of data engineers or data analysts; rather, it’s about enabling data consumers to access and utilize data in a way that aligns more closely with their specific needs. By providing the right tools and access, self-service empowers users to take control of their data-driven processes, reducing bottlenecks, accelerating time-to-insight, and ultimately driving better business outcomes.
Addressing the Delay in Data Access
One of the primary benefits of self-service data is the significant reduction in the time it takes for users to access the data they need. Instead of waiting for data engineers to process and package the data, users can query the source data directly. This is particularly important for data analysts and business users who often need to make quick decisions based on the most up-to-date information. With self-service capabilities, they can access the data in near real-time, allowing them to generate insights more rapidly and respond to changing business conditions with greater agility. This also enables data engineers to spend more time implementing new data sources and systems to accelerate insights instead of being lost in a backlog of requests maintaining existing systems and data.
Reducing the Iterative Cycle
In traditional workflows, the process of gathering requirements, building data models, and delivering dashboards often involves multiple rounds of revisions. Self-service data helps to minimize this iterative cycle by giving users the tools they need to explore data and create the assets they require on their own. By enabling users to experiment with data, create their own queries, and visualize the results, self-service empowers them to refine their requirements and deliver more accurate and relevant insights, all without the need for constant back-and-forth with data engineers.
Lowering the Cost of Revisions
Self-service data also plays a crucial role in reducing the costs associated with revisions. When users can access the data they need directly and make changes to their queries or models on the fly, the need for costly pipeline updates and data extracts is significantly reduced. This not only saves on compute and storage costs but also frees up engineering resources to focus on more strategic tasks, such as optimizing the data infrastructure or developing new features.
Empowering Data Scientists with Agility
For data scientists, self-service data is a quite beneficial. By providing direct access to source data and the ability to create new features on demand, self-service capabilities enable data scientists to iterate on their models more quickly and efficiently. This agility allows them to experiment with different datasets, fine-tune their models, and deliver more accurate predictions without being constrained by the limitations of traditional data pipelines. As a result, organizations can capitalize on new opportunities faster and stay ahead of the competition.
What Self-Service Data Requires
To successfully implement self-service data, organizations need to provide the right combination of technology, governance, and user-friendly interfaces. Self-service doesn’t mean giving users unrestricted access to all data—it means providing secure, governed access to the right data, along with the tools and interfaces that enable users to fulfill their own data needs.
Key Components of a Successful Self-Service Data Platform
Implementing self-service data capabilities requires a thoughtful approach that balances user autonomy with robust governance and security. To empower users while maintaining control over data assets, a self-service data platform must include the following key components:
Secure, Governed Access to Source Data
The foundation of any self-service data platform is secure and governed access to source data. Users need to be able to explore and query data without compromising the integrity or security of the data assets. This requires a platform that can connect to a wide variety of data sources—whether they reside in traditional databases, data warehouses, data lakes, or modern data lakehouse architectures—while enforcing access controls and governance policies.
By providing fine-grained access controls, data engineers can ensure that users only see the data they are authorized to access, while still enabling them to perform meaningful analysis. This balance between access and security is critical to building trust in the self-service platform and ensuring that it is adopted across the organization.
Intuitive User Interface and Tools
Self-service data platforms must be designed with the end user in mind. A key component of this is an intuitive user interface that allows non-technical users to easily interact with data. This includes no-code or low-code tools that enable users to perform complex operations—such as joining tables, filtering data, or creating calculated fields—without writing SQL or relying on a data engineer.
For more advanced users, the platform should also offer powerful SQL capabilities, allowing them to write and execute queries directly. Additionally, features like text-to-SQL, where users can input natural language questions and receive corresponding SQL queries, can significantly lower the barrier to entry for less experienced users, making data more accessible to everyone in the organization.
A Semantic Layer for Consistent, Governed Data Assets
To maximize the value of self-service data, the platform must include a semantic layer that allows users to create, manage, and share data assets in a governed and consistent manner. The semantic layer acts as a bridge between the raw data and the business logic, providing users with a clear, standardized view of the data that aligns with the organization’s data governance policies.
This layer should enable users to document and categorize their data assets, making it easier for others to find and use them. It should also provide mechanisms for tracking changes, managing versions, and rolling back to previous states if necessary. By centralizing the management of data assets, the semantic layer ensures that self-service data activities remain aligned with the organization’s overall data strategy and governance framework.
Monitoring and Auditing Capabilities
Self-service data platforms must also include robust monitoring and auditing capabilities to ensure that data usage aligns with governance policies and to identify any potential issues. This includes tracking who accessed what data, what queries were run, and how data assets were modified. By providing visibility into user activities, the platform enables data engineers and governance teams to proactively manage risks and ensure compliance with internal and external regulations.
How Dremio Facilitates Self-Service Data
Dremio stands out as a leading platform for enabling self-service data, offering a comprehensive set of features that address the challenges of traditional data workflows while empowering users to unlock the full potential of their data. Let’s explore how Dremio’s capabilities align with the key components of a successful self-service data platform.
Direct Access to Source Data with Governance
Dremio’s ability to connect directly to a wide variety of data sources, including databases, data warehouses, data lakes, and data lakehouse catalogs, ensures that users can access the data they need without intermediaries. This direct access is critical for reducing delays and giving users the freshest data possible.
Moreover, Dremio’s platform is built with governance in mind. Data engineers can easily define and enforce access controls, ensuring that users only see the data they are permitted to access. This combination of direct access with strong governance allows organizations to empower their users while maintaining control over their data assets.
User-Friendly Interface with Powerful Tools
Dremio’s user interface is designed to be both powerful and intuitive, catering to users of all technical levels. For business users and analysts, Dremio offers no-code tools like join wizards and commands to change data types, rename columns, and generate calculated fields. These tools make it easy to perform complex data manipulations without writing SQL, enabling users to focus on analysis rather than the mechanics of data processing.
For those who prefer or need to write SQL, Dremio provides a robust SQL editor that supports advanced queries. Additionally, Dremio’s innovative text-to-SQL feature allows users to input natural language questions and receive the corresponding SQL queries, further democratizing access to data.
Integrated Semantic Layer with Git-Like Functionality
Dremio includes an integrated lakehouse catalog that serves as a semantic layer, tracking views and Apache Iceberg tables. This catalog captures the history of data assets at the catalog level, making it easy for data engineers to rollback changes or repair data assets if necessary.
One of Dremio’s standout features is its git-like branching capability. This allows users to create branch environments where they can experiment, make changes, and collaborate without impacting the work of others. Once changes are validated, they can be merged back into the main branch, ensuring that the production environment remains stable. This branching functionality is particularly valuable for data engineers and analysts who need to test new ideas or iterate on data models without disrupting ongoing operations.
Comprehensive Monitoring and Auditing
Dremio’s platform includes comprehensive monitoring and auditing tools that give data engineers and governance teams full visibility into how data is being used. This includes tracking who accessed what data, what queries were run, and how data assets were modified. With these capabilities, organizations can ensure compliance with internal policies and external regulations while also identifying and addressing any potential issues before they escalate.
Conclusion
The journey toward self-service analytics can be challenging, but with the right platform, organizations can overcome the traditional bottlenecks and inefficiencies in data workflows. Dremio provides a powerful, user-friendly, and secure platform that enables organizations to deliver on the promise of self-service data. By empowering users with direct access to data, intuitive tools, and robust governance, Dremio not only accelerates time-to-insight but also reduces costs and enhances data-driven decision-making.
As organizations continue to navigate the complexities of modern data environments, Dremio’s self-service capabilities offer a clear path forward, allowing businesses to unlock the full value of their data assets while maintaining control and governance. With Dremio, the future of self-service analytics is not just achievable—it’s within reach.
Setup a meeting with us today to explore how Dremio can provide self-service to your organization, and feel free to hands-on with Dremio for free today!
Sign up for AI Ready Data content