7 minute read · December 3, 2020
Predictions 2021: Five Big Data Trends You Should Know
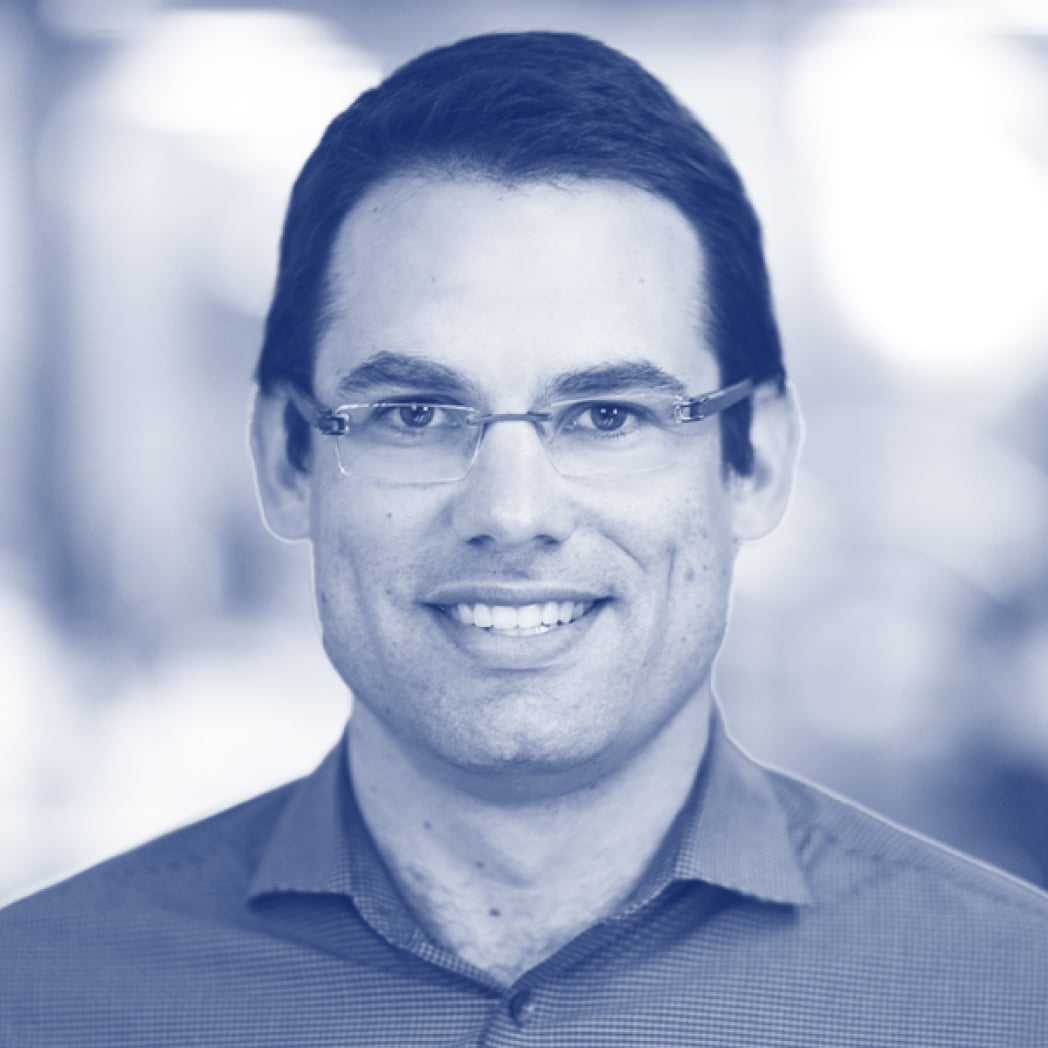
· Founder & Chief Product Officer, Dremio
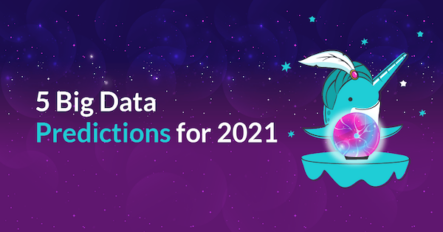
In 2020 we experienced unprecedented market shifts that required data and analytics leaders to quickly adapt to the increasing velocity and scale of data. In 2021, many organizations will look beyond any short-term fixes and implement modern data architectures that both accelerate analytics and keep costs under control. The companies that accomplish both of these will outpace their competition.
Five major trends will emerge in the new year that bring compelling reasons to make modern cloud data lakes the center of gravity for data architectures. They challenge a 30-year-old paradigm that, in order to query and analyze data, data teams need to extract and load it into a costly, proprietary data warehouse. Combined with the increased need for cost control, security and data governance, this will also drive a shift in power to centralized data teams.
Trend #1: Separation of Compute and Data Becomes the Default Choice
For years, the industry has talked about the separation of compute and storage. However, it is only with the widespread adoption and migration to public clouds that it has become a reality. The separation of compute and storage provides efficiencies that were not possible in architectures that co-located compute and storage, such as on-premises data warehouses and Hadoop clusters. In the coming year, however, another paradigm for fully leveraging cloud infrastructure resources will emerge—one that puts data at the center of the architecture.
The rise of cloud data lake storage (e.g., Amazon S3 and Azure Data Lake Storage) as the default bit bucket in the cloud, combined with the infinite supply and elasticity of cloud compute resources, has ushered in a new era in data analytics architectures. Just as applications have moved to microservice architectures, data itself is now able to fully exploit cloud capabilities. Data can be stored and managed in open source file and table formats such as Apache Parquet and Apache Iceberg, and accessed by decoupled and elastic compute engines such as Apache Spark (batch), Dremio (SQL) and Apache Kafka (streaming). With these advances data will, in essence, become its own tier, enabling us to rethink data architectures and leverage application design benefits for big data analytics.
Trend #2: The Shine of the Cloud Data Warehouse Wears Off
The cloud data warehouse vendors have leveraged the separation of storage from compute to deliver offerings with a lower cost of entry than traditional data warehouses, as well as improved scalability. However, the data itself isn’t separated from compute — it must first be loaded into the data warehouse and can only be accessed through the data warehouse. This includes paying the data warehouse vendor to get the data into AND out of their system. So, while upfront expenses for a cloud data warehouse may be less, the costs at the end of the year are likely significantly higher than expected.
Meanwhile, low-cost cloud object storage is increasingly making the cloud data lake the center of gravity for many organizations’ data architectures. While many data warehouses provide a mechanism to query the data in the data lake directly, the performance isn’t sufficient to meet business needs. As a result, even if they are taking advantage of the low-cost cloud data lake storage, organizations still need to copy and move data to their data warehouse and incur the associated data ingest costs. By leveraging modern cloud data lake engines and open source table formats like Apache Iceberg and Project Nessie, however, companies can now query data in the data lake directly without any degradation of performance, resulting in an extreme reduction in complex and costly data copies and movement.
Trend #3: The Data Lake Can Do What Data Warehouses Do and Much More
Enterprise data warehouses offer key capabilities for analytics workloads beyond querying the data, such as data mutations, transactions and time travel. However, they do so through a closed, vertically integrated and proprietary system where all access must go through and be processed by the database. Routing all access through a single system simplifies concurrency management and updates but also limits flexibility and increases cost.
A new open source table format, Apache Iceberg, solves these challenges and is rapidly becoming an industry standard for managing data in data lakes. It provides key data warehouse functionality such as transactional consistency, rollbacks and time travel while introducing new capabilities that enable multiple applications to work together on the same data in a transactionally consistent manner. As a result, all applications can directly operate on tables within data lake storage. Doing so not only lowers cost by taking advantage of data lake architectures but also significantly increases flexibility and agility since all applications can work on datasets in place without migrating data between multiple separate and closed systems.
Another new open source project, Project Nessie, builds on table formats like Iceberg and Delta Lake to deliver capabilities not available in data warehouses today. It provides a Git-like semantics for data lakes which enables users to take advantage of branches to experiment or prepare data without impacting the live view of the data. In addition, Nessie makes loosely-coupled transactions a reality, enabling a single transaction spanning operations from multiple users and engines including Spark, Dremio, Kafka and Hive. It also makes it possible to query data from consistent points in time as well as across different points in time, making it easier to reproduce results, understand changes and support compliance requirements.
Trend #4: Data Privacy and Governance Kicks Into Another Gear in the United States
Users are increasingly concerned about their online privacy making it much more likely that the United States will adopt regulations similar to Europe’s General Data Protection Regulation (GDPR) and the California Consumer Privacy Act (CCPA). This will require companies to double down on privacy and data governance in their data analytics infrastructure. Furthermore, companies will realize that data privacy and governance cannot be achieved with separate standalone tools, and instead must be implemented as an integral part of the analytics infrastructure.
In 2021, data warehouses and data lakes will begin to provide such capabilities within their platforms. Data version control will become standard in cloud data lakes. Open source technologies such as Project Nessie will enable companies to securely manage and govern data in an enterprise-wide platform.
Trend #5: Increased Need for Cost Control and Data Governance Shifts the Power Back to Centralized Data Teams
The increasing demand for data and analytics means that data teams are struggling to keep up with never-ending requests from analysts and data scientists. As a result, data is often extracted and shared without IT’s supervision or control. Furthermore, the scramble to meet the query performance requirements of these data consumers has resulted in many copies and permutations of the data floating around. This results in runaway costs as well as data governance challenges.
At the same time, macro-economic conditions combined with new privacy laws and breach concerns will shift power back to centralized data teams. These teams will invest in building enterprise-wide data platforms such as cloud data lakes, allowing them to drastically reduce overall cloud costs by eliminating data copies and the need to leverage expensive data warehouses. Furthermore, the ability to modify datasets and delete records directly within data lakes will make it easier to handle the right to be forgotten, while open source data version control technologies such as Project Nessie will enable centralized data governance by eliminating silos and promoting data integrity.
Learn More
To learn more about my predictions for 2020, please check out this webinar.