4 minute read · March 26, 2020
The COVID-19 Paradox: Advancing Your Data Analytics Programs in the Midst of a Pandemic

· Founder & Chief Product Officer @ Dremio
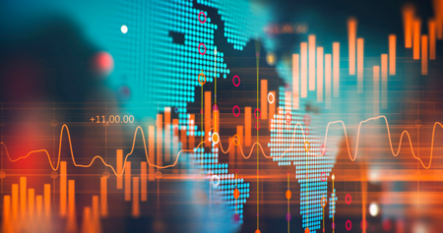
It’s 2020, and almost every organization is facing a paradox. On one hand, data is an integral part of the business, and analytics has become a strategic priority - a “must-have.” A recent article from McKinsey stressed the importance of continuing to invest in strategic programs like data and cloud in times like this. On the other hand, the economic impact of COVID-19 has forced almost every organization to reduce spending, making it challenging to invest in expensive technologies such as cloud data warehouses and resource-hungry compute engines.
When we founded Dremio 4.5 years ago, we set out on a mission to empower every company in the world to become data driven. Needless to say, we did not envision the current situation. Today, as we announce our $70M series C in the midst of a very challenging time for organizations around the world, we feel an even greater sense of responsibility and commitment to our mission. We believe, now more than ever before, that the technology we have developed over the last four years, and the investments that we are making today, will enable organizations to continue advancing their strategic data initiatives because we enable them to do so with a drastically lower cost structure.
How is this possible? There are two major trends that are enabling a much more scalable and cost-efficient approach to data analytics:
- The rise of data lake storage - S3 and ADLS. For many organizations, cloud data lake storage can even be viewed as the de-facto system of record in the cloud.
- The adoption of a new architectural paradigm defined by loosely-coupled services and systems. O’Reilly calls this the Next Architecture.
The promise of the cloud data lake
Amazon.com, a company that has been defined by cost efficiency perhaps more than any other, is embracing this new approach. Werner Vogels, the company’s CTO, recently blogged about how Amazon built a strategic company-wide data lake on S3 to break down silos and empower the various teams across the company to analyze data. In this post, he identified numerous challenges that a cloud data lake helps them solve. For example:
- Data silos. “Having pockets of data in different places, controlled by different groups, inherently obscures data … A data lake solves this problem by uniting all the data into one central location. Teams can continue to function as nimble units, but all roads lead back to the data lake for analytics. No more silos.”
- Inconsistent semantics. “Different systems may also have the same type of information, but it’s labeled differently. For example, in Europe, the term used is ‘cost per unit,’ but in North America, the term used is ‘cost per package.’ The date formats of the two terms are also different.”
- No centralized security and governance. “Amazon’s operations finance data is spread across more than 25 databases with regional teams creating their own local version of datasets. … Audits and controls must be in place for each database to ensure that nobody has improper access.”
These challenges are, of course, hard to solve with a data warehouse. According to Vogels, “If you wanted to combine all of this data in a traditional data warehouse without a data lake, it would require a lot of data preparation and export, transform, and load (ETL). You would have to make trade-offs on what to keep and what to lose and continually change the structure of a rigid system.”
As you can see, cloud data lakes are much more scalable and cost-efficient than data warehouses. However, in order for most organizations to realize the value, they need to (1) become a lot easier to build and manage (i.e., most companies don’t have Amazon’s engineering resources); and (2) address the needs of non-technical users (i.e., those who use Tableau and Power BI). For example, BI users that have tried to directly analyze data residing in data lake storage with SQL engines (e.g., Hive, Presto, Athena) have had limited success. The performance is simply inadequate (sometimes by orders of magnitude), the data is too messy, and the infrastructure is prohibitively expensive. These are the exact problems that we are solving at Dremio. In Part 2, we will discuss how we are doing that, and what’s in store for the future.
Sign up for AI Ready Data content