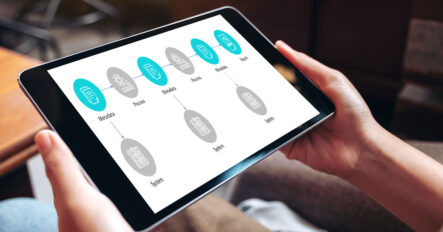
Data Lineage Definition:
Data lineage refers to the data’s “line of descent.” In other words, it’s a record of how data got to a specific location and the intermediate steps and transformations that took place as it traveled through business systems. For organizations that depend on data, understanding where data comes from, evaluating its quality, and determining its accuracy are essential to supporting the business. Data lineage essentially provides a map of the data journey that includes all steps along the way, as illustrated below:

“Data lineage is a description of the pathway from the data source to their current location and the alterations made to the data along the pathway.”
— Data Management Association (DAMA)
As data explodes in velocity, variety and veracity, it is important to track the lineage of the data — how it is transformed. Data lineage answers questions such as:
- Where did the data originate?
- What kind of transformations did the data go through?
- Where did the data land?
Why Does Data Lineage Matter?
Understanding the provenance and lineage of data sources is valuable for several reasons:
- Evaluating the trustworthiness of data based on its provenance
- Understanding and correcting sources of error
- Identifying incorrect assumptions about data that may skew analysis
- Providing audit trails for data governance and regulatory purposes
- Ensuring data flows are protected and not subject to tampering
- Identifying and avoiding data duplication to simplify operations and reduce cost
Organizations need visibility to how information moves through various workflow steps to ensure the quality of query results, business reports, business intelligence (BI) dashboards and training sets. Data quality is enhanced when data engineers can track who made a change and why, how something was updated, and which process was used.
Business Value of Data Lineage
While data lineage may seem like an abstract concept, having full visibility to data through its lifecycle can bring value to the business across multiple areas. Some of the key benefits of data lineage management include:
Trustworthiness
Data lineage helps you evaluate the trustworthiness of data based on its provenance.
Audit and Compliance
Data lineage provides audit trails for data governance and regulatory purposes and reduces the cost of compliance with existing and future regulation.
Cloud Migration
Data lineage helps you migrate data to the cloud by providing context that enables impact analysis. With data lineage, you can plan your migration and ensure that there’s no impact on downstream data consumers.
Visibility for Data Users
Data lineage provides visibility for self-service analysis by business users – which includes visibility into how data moves and transforms through the data pipelines, business reports, business intelligence dashboards.
Data Quality
Data lineage offers better quality data for better analysis and business results and helps avoid data duplication. It can also help you understand and correct data errors.
Business Agility
Data lineage can help you build an agile data infrastructure that is responsive to change and reduces the cost of application maintenance and new development.
Reduce IT Cost and Risk
Modern enterprises rely on BI and decision support systems (DSS) for almost every decision they make. Examples include features to prioritize in new product designs, where to place advertisements, and what sales and marketing strategies to employ to maximize revenue, profitability and customer loyalty. Getting the data wrong can seriously skew the results and undermine business performance.
Better Handling of Evolving Data Sources
As business conditions evolve, systems and data sources change constantly. For example, an analytic application that evaluates customer behavior by looking only at traditional point of sale data is almost sure to be wrong. This method of analysis will have missed e-commerce orders, in-app purchases, and customers across a variety of other sales channels and demographics. While this seems obvious, data bias and undetected problems with data sources is a problem that even the most sophisticated organizations can easily fall into.
Managing Data Lineage
In data lake environments, managing data lineage is especially critical. Data lakes contain diverse datasets, in different formats that come from a wide variety of sources. For example, data lakes may contain images, video files, log files, documents, raw text or files in formats such as JSON, CSV, Apache Parquet or Optimized Row Columnar (ORC) formats. Also, datasets in the data lake are continually being added to, often at a rapid pace, and a variety of tools may access and process this raw data, resulting in additional derivative datasets.
When these issues of variety and velocity are coupled with the sheer volume of data, it becomes impossible for individuals to manually track the origins and details about every data item. In data lake environments, metadata management must be automated. When managing data lakes, metadata management is a particular concern. Unlike the data itself stored in the data lake, metadata is “the data about the data.” Metadata can take a variety of forms. For example, technical metadata may contain supplementary information about the data type, format and structure (schema). Business metadata may contain information about business objects and descriptions. Operational metadata typically contains information about data processing critical to tracking data lineage.
Next-Generation Data Lineage: Why it Matters
Data engineers and data architects need metadata management tools to manage data lakes and enable self-service access. A next-generation metadata management solution creates a business-friendly semantic layer on top of physical data in the data lake, enabling data consumers to view data lineage and interact with data in the lake without having to rely on IT.
For example, Dremio’s semantic layer exposes a business representation of an organization’s data assets so that it can be accessed using common business terms. This semantic layer creates an abstraction layer so that business users can interact with the objects created in it without worrying about the complexities of where and how the data is physically stored and organized.
Ideally, a metadata management solution should provide capabilities such as a standard interface to query data in the data lake, access control, data masking for sensitive information, data encryption, and auditing to track the integrity of data assets and track lineage throughout the data lifecycle.
Sign up for AI Ready Data content