6 minute read · July 16, 2019
Four Key Elements to Designing a Successful Data Lake – Dremio
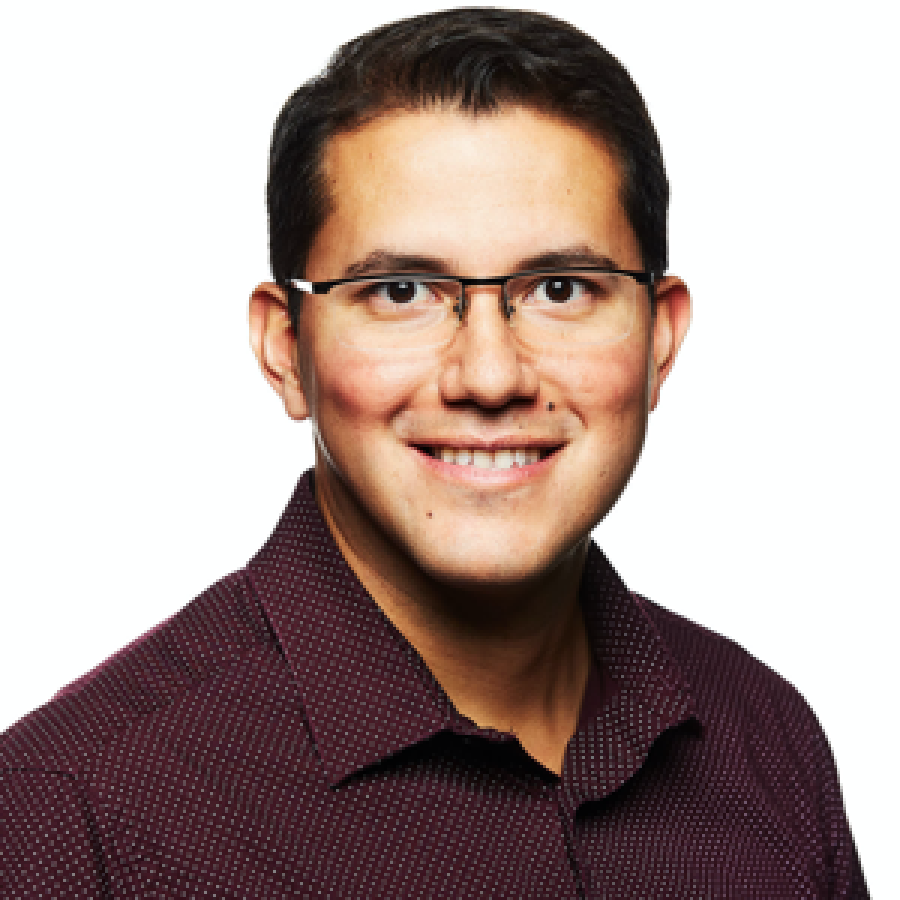
· Director of Technical Marketing, Dremio
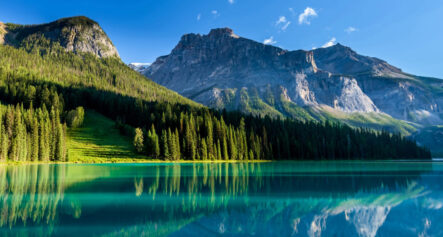
Discovering insights from data lakes can be challenging.
A data lake is a system used to store data for analytics. Cloud services like Azure Data Lake Store (ADLS) and Amazon S3 are examples of a data lake, as is, the distributed file system used in Apache Hadoop (HDFS). Companies that store large amounts of data build data lakes for their flexibility, cost model, elasticity, and scalability.
Yet the data in data lakes tends to be less structured and less understood, and for data consumers to analyze the data in the data lakes, IT needs to move data to a data warehouse and then build extracts and cubes. The burden for understanding and structuring the data then falls on IT, the data engineers who take months to build pipelines so that the data can be accessed, and it also falls on the data consumers, who need to wait for IT so that they run their BI workloads.
When BI users have access to the data, they still need to prepare, catalog, and accelerate their data, and they don’t have an easy interface or access point for them to do so. This process adds costs and complexity and delays access to what is most crucial—your data. Lastly, companies don’t understand exactly what their data lake contains or the quality of their data.
Data lakes are an agile, low-cost way for companies to store their data, but without the right tools, the data lake can grow stagnant and become a data swamp. Often, data lakes suffer or fail when there is no way to govern the data, no easy way for data consumers to access the data, and no clear goal for what it is supposed to achieve.
The four keys to success
When designing a data lake, there are four important considerations to make it accessible for data consumers.
Data acquisition
When data is sourced for the data lake, schema and data quality must be a priority so the data lake can be usable for data consumers.
Data curation
Data consumers need to be able to use their favorite tools, such as Tableau, Python, and R. Typically a data lake is used to store raw datasets, and then vetted data is moved into a data warehouse for access.
Optimization and governance
Once insights have been made, the process must be streamlined so that it is ready for enterprise-level outputs, often requiring data preparation or transformation, a data catalog or semantic layer, query acceleration, robust data integration, and data governance to ensure data quality.
Analytics consumption
Data consumers need capabilities such as the ability to run ad hoc queries, low latency, high concurrency, workload management, and integration with BI tools. Data lakes do not provide this functionality, making it difficult for end users to work within the data lake environment.
Dremio allows you to wade through the complexities of your data lake
Dremio connects to data lakes like ADLS, Amazon S3, and more, putting all of your data in one place and providing it structure. We provide an integrated, self-service interface for data lakes, designed for BI users and data scientists. Dremio increases the productivity of these users by allowing them to easily search, curate, accelerate, and share datasets with other users. In addition, Dremio allows companies to run their BI workloads from their data lake infrastructure, removing the need to build cubes or BI extracts.
Dremio helps you leverage your data lake
Data acquisition
With Dremio, you don’t need to worry about the schema and structure of the data that you put in your data lake. Dremio takes data from whatever kind of source–relational or NoSQL–and converts it into a SQL-friendly format without making extra copies. You can then curate, prepare, and transform your data using Dremio’s intuitive user interface, making it ready for analysis.
Data curation
Dremio makes it easy for your data engineers to curate data for the specific needs of different teams and different jobs, without making copies of the data. By managing data curation in a virtual context, Dremio makes it fast, easy, and cost effective to design customized virtual datasets that filter, transform, join, and aggregate data from different sources. Virtual datasets are defined with standard SQL, so they fit into the skills and tools already in use by your data engineering teams.
Optimization and governance
In order to scale these results across your enterprise, Dremio provides a self-service semantic layer and governance for your data. Dremio’s semantic layer is an integrated, searchable catalog in the Data Graph that indexes all of your metadata, allowing business users to easily make sense of the data in the data lake. Anything created by users—spaces, directories, and virtual datasets make up the semantic layer, all of which is indexed and searchable. The relationships between your data sources, virtual datasets, and all your queries are also maintained in the Data Graph, creating a data lineage, allowing you to govern and maintain your data.
Analytics consumption
At its core, Dremio makes your data self-service, allowing any data consumer at your company to find the answers to your most important business questions in your data lake, whether you’re a business analyst who uses Tableau, Power BI, or Qlik, or a data scientist working in R or Python. Through the user interface, Dremio also allows you to share and curate data virtual datasets without making extra copies, optimizing storage and supporting collaboration across teams. Lastly, Dremio accelerates your BI tools and ad-hoc queries with reflections, and integrates with all your favorite BI and data science tools, allowing you to leverage the tools you already know how to use on your data lake.