Apache Iceberg has been the focus of a lot of discussions recently on the topic of turning data lakes into data lakehouses and reducing the data warehouse footprint. Mainly this has been in response to many issues with modern data warehouses, including:
- Regulatory risk: Data drift caused by many scattered copies of data across the data lakes, data warehouses, data marts, cubes and BI extracts can lead to difficulty in maintaining compliance with regulatory data retention and disposal requirements.
- Inconsistency: Having so many scattered copies of the data leads to inconsistent data and inconsistent insights.
- Lack of self-service: Due to the several steps required to get data into the warehouse, data becomes less self-service. This puts pressure on the data engineering team to handle constant requests to import data not yet in the warehouse along with creating new data marts, cubes, and extracts to facilitate consumer demands.
- Lock-in: When your data is in a data warehouse and you want to migrate off that system (i.e., for cost reasons), it’s very challenging, time-consuming, and costly to migrate out of the system successfully.
- Lock-out: Having your data in a walled garden like a data warehouse makes it harder to take advantage of the latest innovations in data because your data may not be able to be accessed by external tools.
- Costs: Data warehouses are notoriously expensive in general, and you also pay for unnecessary compute and storage — ETL compute to ingest the data and storage to store the data in the data warehouse in addition to the existing storage in the data lake.
To better understand how Apache Iceberg can be the solution, let’s first examine the problem.
Understanding the Status Quo
In traditional architecture, you follow a particular pattern:
- You export your data from your data sources such as traditional databases, and land them in a data lake. You incur costs for the compute to carry out the ETL job and the storage of your new analytical copy of the data.
- You then export data from your data lake into a data warehouse, making an additional copy that incurs more compute costs in transferring the data and more storage costs to store it.

When following this pattern you run into many of the problems with data warehouses mentioned earlier:
- Regulatory risk
- Inconsistency
- Lack of self-service
- Lock-in
- Lock-out
- Costs
You can address these challenges by running all your data warehouse workloads directly on your data lake to minimize the data warehouse footprint.
- Regulatory risk: With fewer copies, there is less to monitor and lower likeliness of regulatory noncompliance and the possible fines that come with it.
- Inconsistency: With fewer copies, the data lake acts as the consistent “source of truth.”
- Lack of self-service: By letting consumers work directly on the lake, they have access to more data using tools like Dremio that they can curate themselves for more self-service access to the data.
- Lock-in: Your data is never stored in a walled garden as it is under your control, in your storage, and in open formats. If using a particular tool or service gets too expensive, you can make a switch without moving data.
- Lock-out: As new tools and platforms offer new value propositions, you can try them out or fully adopt them without having to worry about elaborate data migrations.
- Costs: Without having to ETL and store your data twice for a data warehouse you can lower your costs, and with the right tools and formats you can also significantly improve the cost of running your queries.
For this to happen, an abstraction layer is needed that allows for tooling to see the sea of files stored in the data lake as traditional tables. Note this is something data warehouses also do, but is hidden underneath proprietary layers.
To solve this problem the original table format for data lake 1.0 was created via the Apache Hive project but came with several challenges:
- Small updates are very inefficient
- No way to change data in multiple partitions safely
- In practice, multiple jobs modifying the same dataset don’t do so safely
- All of the directory listings needed for large tables take a long time
- Users have to know the physical layout of the table
- Table statistics are often stale
These challenges resulted in the inability of the data lake to fulfill the desire to replace the data warehouse.
A better solution was needed to enable these workloads to be run on the data lake.
Two Birds, One Stone
In the status quo, you get different benefits from your data lake and data warehouse. The data lakehouse provides the benefits of both worlds:
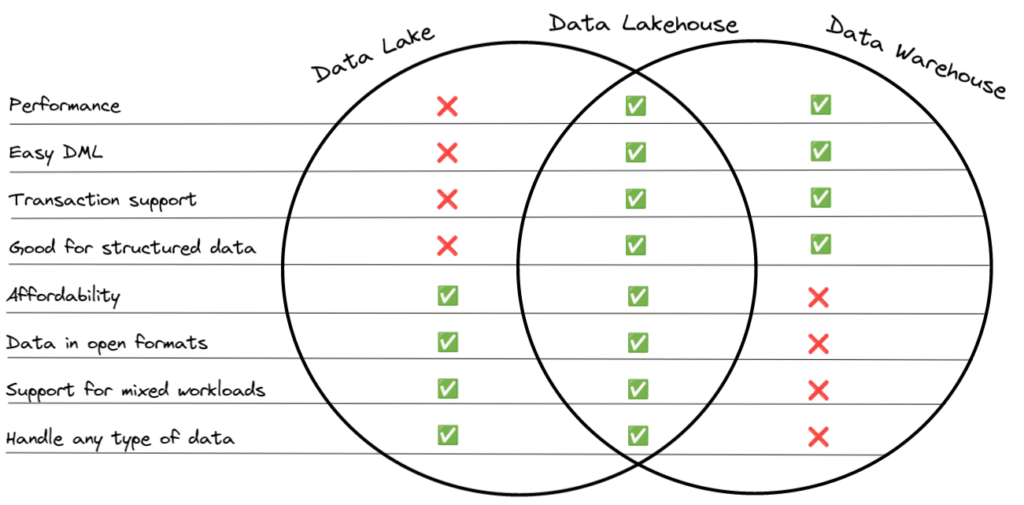
Data lakehouse architecture mitigates the cons of both data lakes and data warehouses and combines the pros:
- By working directly on the data in the data lake you reduce the need for duplicative storage and compute
- Using table formats like Apache Iceberg you enable performance, DML, and transactions
- Using open file formats like Apache Parquet and open table formats like Apache Iceberg, any engine or tool can be used on the data — both today (e.g., for different business units wanting to use different tools) and tomorrow (e.g., a new tool comes out that a business unit wants to use)
- Using data lakehouse platforms like Dremio you can access data from many sources and provide consumers an easy-to-use interface
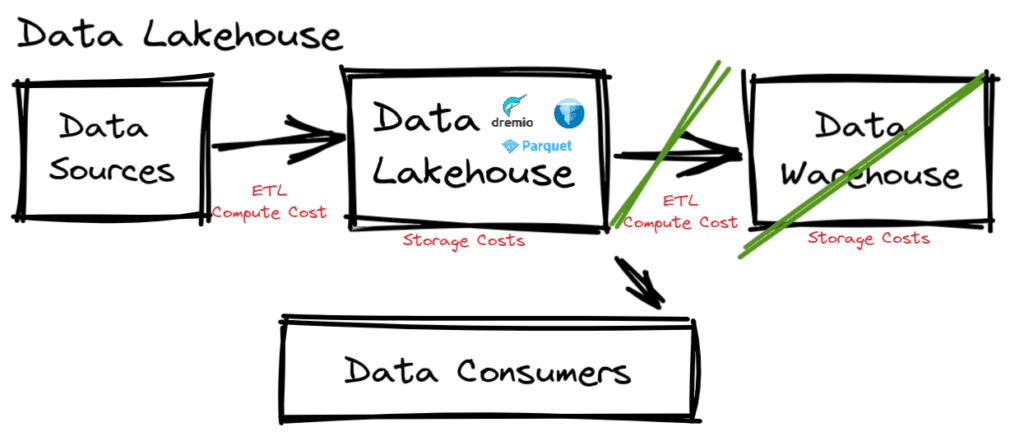
So you want to implement a data lakehouse, but the Hive table format doesn’t allow you to achieve the promise of a data lakehouse. This is where Apache Iceberg becomes the key piece in your data architecture.
Apache Iceberg is a table format that addresses the challenges with Hive tables, allowing for capabilities like ACID transactions, time-travel, and table evolution, truly enabling those warehouse-like features in a data lakehouse. By making a data lakehouse possible and practical, you can now begin to eliminate many of the problems with the traditional approach.
The Tip of the Iceberg
Apache Iceberg isn’t the only table format competing to be the cornerstone of the modern data lakehouse, although Apache Iceberg has a lot of unique value propositions:
- Apache Iceberg is the only table format with partition evolution which allows you to evolve your table partitioning without expensive table rewrites.
- Apache Iceberg’s hidden partitioning feature makes it much easier for data consumers to take advantage of table partitioning, resulting in higher performance, reduced compute, and lower cost of the data platform.
- Apache Iceberg’s object store file layout and overall decoupling from Hive’s file structure allows for better performance on object storage at any scale.
- Apache Iceberg offers the ability to fine-tune row-level operations (Copy-on-Write vs. Merge-on-Read) not only at the table level but also by type of operation (merge, update, delete).
- Apache Iceberg has a robust ecosystem of users, developers, and compatible tools for querying, ETL, and more.
Project Nessie is an open-source project that provides catalog versioning, providing Git-like capabilities for Apache Iceberg tables that enables patterns like isolating ETL work and multi-table transactions (committing changes to multiple tables simultaneously).
Conclusion
Reducing your data warehouse footprint with an Apache Iceberg-based data lakehouse will open up your data to best-in-breed tools, reduce redundant storage/compute costs, and enable cutting-edge features like partition evolution/catalog branching to enhance your data architecture.
In the past, the Hive table format did not go far enough to make this a reality, but today Apache Iceberg offers robust features and performance for querying and manipulating your data on the lake.
Now is the time to turn your data lake into a data lakehouse and start seeing the time to insight shrink along with your data warehouse costs.