Data Lakes Drive Decisions: A Virtual Fireside Chat
Join us for a fireside chat with Mai-Lan Tomsen Bukovec, the global Vice President for Amazon Web Services (AWS) Block and Object Storage services, which include Elastic Block Storage (EBS), Simple Storage Service (S3) and Amazon Glacier. S3 has more data lakes than any other provider, and Mai-Lan will discuss emerging trends in data lakes and how they are powering the next generation of business analytics, machine learning and other business-critical applications.
Topics Covered
Speakers
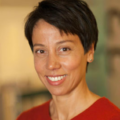
Mai-Lan Tomsen Bukovec
Mai-Lan Tomsen Bukovec is the global Vice President for Amazon Web Services (AWS) Block and Object Storage services, which include Elastic Block Storage (EBS), Simple Storage Service (S3) and Amazon Glacier. Mai-Lan has been an engineering and product leader of AWS storage and compute services since 2010. Prior to joining Amazon, Mai-Lan spent over 10 years in engineering and product leadership roles at Microsoft, as well as three years in early stage startups. She served as a forestry volunteer in the Peace Corps in Mali, West Africa.
Mai-Lan lives in Seattle with her husband and three boys. When she is not working on Amazon cloud services and spending time with her family, Mai-Lan trains as a recreational boxer. She also holds a green glove ranking in the martial art Savate.
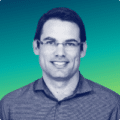
Tomer Shiran
Tomer Shiran is the CPO and founder of Dremio. Prior to Dremio, he was VP Product and employee #5 at MapR, where he was responsible for product strategy, roadmap, and new feature development. As a member of the executive team, Tomer helped grow the company from five employees to over 300 employees and 700 enterprise customers. Prior to MapR, Tomer held numerous product management and engineering positions at Microsoft and IBM Research. He holds a master’s degree in electrical and computer engineering from Carnegie Mellon University and a bachelor’s in computer science from Technion – Israel Institute of Technology, as well as five U.S. patents.
Sign up for AI Ready Data content