High Frequency Small Files vs. Slow Moving Datasets
Before implementing Apache Iceberg, we had a small file problem at Adobe. In Adobe Experience Platform’s (AEP) data lake, one of our internal solutions was to replicate small files to use at a very high frequency of 50K files per day for a single dataset. A streaming service we called Valve processed those requests in parallel, writing them to our data lake and asynchronously triggering a compaction process. This worked for some time but had two major drawbacks. First, if the compaction process was unable to keep up, queries on the data lake would suffer due to expensive file listings. Second, with our journey with Iceberg underway, we quickly realized that creating thousands of snapshots per day for a single dataset would not scale. We needed an upstream solution to consolidate data prior to writing to Iceberg.In response, a service called Flux was created to solve the problem of small files being pushed into a slow-moving tabular dataset (aka Iceberg v1). In this presentation, we will review the design of Flux, its place in AEP’s data lake, the challenges we had in operationalizing it and the final results.
Topics Covered
Speakers
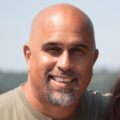
Shone Sadler
Shone Sadler is a Principal Data Scientist at Adobe Systems working on the Adobe experience platform.
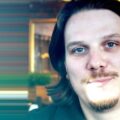
Andrei Ionescu
Andrei Ionescu is a Senior Software Engineer with Adobe, and he is part of Adobe Experience Platform’s Data Lake team, specializing in big data and distributed systems with Scala, Java, Spark, and Kafka. At Adobe, he is mainly contributing to ingestion and data Lake projects, while on open source he is contributing to Hyperspace and Apache Iceberg.
Sign up for AI Ready Data content