TDWI – Unlocking the Potential of Data Lakehouses: AI, Data Mesh, and Survey Insights, Part 2 of 2
More organizations are seeking a competitive edge by using technology such as AI to help them make more informed decisions, enhance operational efficiency, and drive innovation. As part of this evolution, enterprises are using high volumes of diverse data, resulting in a complex data landscape. Data professionals are taking greater interest in cloud data lakehouses as a solution for modern data management challenges. Data warehouses were designed for structured data, data lakes for managing large volumes of unstructured data. The lakehouse combines the scalability of data lakes and the reliability and performance of data warehouses.
At the same time, organizations are adopting new frameworks such as data mesh to overcome challenges posed by the complexity of modern AI data ecosystems. How are organizations utilizing data lakehouses for AI and how are they implementing a data mesh paradigm? In part two of this two-part series, Dremio’s CMO, Read Maloney, will share the results of the company’s survey of 500 organizations about AI, data mesh, and the data lakehouse. TDWI’s VP of Research, Fern Halper, Ph.D., will share TDWI research on the topic. They will discuss how organizations are using the lakehouse for AI and how data mesh helps enable scalability and agility and improve decision-making.
Watch and learn about:
- The current state of data lakehouse adoption
- How the data lakehouse is being used for AI
- Why and how organizations are implementing a data mesh framework to support AI on the lakehouse
- Best practices for getting started with AI and data mesh on the lakehouse
Register to view webinar
Speakers
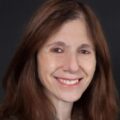
Fern Hapler
Fern Halper, Ph.D., is vice president and senior director of TDWI Research for advanced analytics. She is well known in the analytics community, having been published hundreds of times on data mining and information technology over the past 20 years. Halper is also co-author of several Dummies books on cloud computing and big data. She focuses on advanced analytics, including predictive analytics, social media analysis, text analytics, and big data analytics approaches. She has been a partner at industry analyst firm Hurwitz & Associates and a lead analyst for Bell Labs. Her Ph.D. is from Texas A&M University.
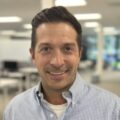
Read Maloney
Read is a cloud, data, and AI marketing executive, with a history of building and leading high-growth marketing teams at AWS, Oracle, and H2O.ai. Most recently at H2O.ai, he served as the SVP of Marketing, leading all elements of marketing for the late-stage startup. Prior to working in the technology industry, Read was a Captain in the United States Marine Corps, serving two tours of duty as a Platoon Commander in Iraq. Read holds a Bachelor’s Degree in Mechanical Engineering from Duke University and an M.B.A from the Foster School of Business at the University of Washington.
Transcript
Note: This transcript was created using speech recognition software. While it has been reviewed by human transcribers, it may contain errors.
Opening
Andrew Miller:
Hello, everyone, and welcome to the TDWI Webinar program. I'm Andrew Miller, and I'll be your moderator. For today's program, we're going to talk about unlocking the potential of data lakehouses with AI, data mesh, as well as survey insights. This is part 2 of our series. Our sponsor today is Dremio.
For our presentation, today we'll hear from Fern Halper with TDWI, and Read Maloney with Dremio again. Today we're going to be discussing how to unlock the potential of data lakehouses, and our first speaker is Fern Halper. She's the Vice President and Senior Director of TDWI Research for Advanced Analytics. Fern is well known in the analytics community, having been published hundreds of times on data mining and information technology over the past 20 years. She is also the co-author of several Dummies books on cloud computing and big data. Fern focuses on advanced analytics, including predictive analytics, machine learning, AI, cognitive computing, and big data analytics approaches. She's been a partner at industry analysts firm, Hurwitz and Associates, and a lead data analyst for Bell Labs. Fern has also taught at both Colgate University and Dental University, and her PhD is from Texas A&M University. Our guest speaker today is Read Maloney, with Dremio. Read is the CMO of Dremio and is a Cloud Data and AI marketing executive, with a history of building and leading high-growth marketing teams at AWS, Oracle, and H2o.ai. Most recently at H2o.ai, he served as the Senior Vice President of marketing, leading all elements of marketing from the late-stage startup. [Before] working in the technology industry, Read was a captain in the United States Marine Corps, serving 2 tours of duty as a platoon commander in Iraq. Read holds a bachelor's degree in mechanical engineering from Duke University and an MBA from the Foster School of Business at the University of Washington. Welcome both Fern and Read. And with that, I'll hand it over to you, Fern, for your presentation.
Topics
Fern Halper:
Thanks, Andrew. Hi, everyone, and welcome to the second in a 2-part series on the Data Lakehouse, so we're glad you could join us today. We're going to talk about data mesh, AI, and the lakehouse. So if you were on our previous webinar, you'll know that we're doing things a little differently in these kinds of webinars––we're going to share the results not only of TDWI research but also a study that Dremio commissioned about the state of the Data Lakehouse. We'll talk about why organizations are implementing a data mesh paradigm with the lakehouse to support AI and how the lakehouse is being used. So I'm going to kick things off, I'm going to talk about the state of the Data Lakehouse in terms of what TDWI is seeing, and what we're seeing in terms of data mesh, and then Read will share Dremio's findings. And then I'll talk about AI, and we'll go back and forth is what's going to happen here.
Data Lakehouse Definition
Fern Halper:
So first, let me define what we mean when we say a data lakehouse. This is a platform that's a combination of a data lake and a data warehouse that provides warehouse data structures and data management functions on a low-cost platform such as a cloud object store. I have heard that these new platforms have blurred the distinction between traditional data warehouses and data lakes––they support large volumes of diverse data, along with SQL, BI, AI, machine learning, and other advanced analytics on one unified common platform—and this is most often in the cloud. I'd say that the Data Lakehouse concept grew out of the fact that organizations need to collect and manage diverse data types, so we're not just talking about structured data here, but also unstructured data, such as text and images. And organizations use their data warehouse for structured data, and then the data lake for unstructured data. But as many of these data lakes evolved, they evolved into large data storage platforms that weren't performant. They weren't organized. They just weren't useful; they weren't necessarily high-quality data platforms, let's put it that way. And that led to this notion of a data lakehouse.
Data Lakehouses in Use and Expected to Grow
Fern Halper:
Here is data from a 2023 TDWI survey that shows you the lakehouse adoption and how it's set to grow. And so here, it’s a long survey question we asked, which of the following types of data management platforms, repositories, or patterns are currently in use or planned for use by your organization to support BI analytics, AI, etcetera? And so the dark gray here is currently using the dark blue is planning to use; the light blue is not planning, and you could see there's another light blue under there, but just pay attention to the dark gray and the darker blue, and you could see in the survey that we have data warehouses on-premises, and those are slightly beating out the data warehouse on the cloud, but that more people were planning on cloud implementations. I've typically seen now that cloud platforms are outpacing platforms on-premises. And then, if you look down there, I have circled something that's called the Unified Data Warehouse-Data Lake platform, eg. the Cloud Data Lakehouse. And that one was at about 20-something percent using it now, which is pretty amazing, considering when it was introduced. And then 46% were planning to make use of it. And if you look at that dark blue bar that's bigger than any other platform type listed here––so those making use of data lakehouses still make use of other platforms, but they're less likely to make use of an on-premises data warehouse. So there's a great deal of interest and adoption occurring for data lakehouses. Why are they using the data lakehouse? Because organizations don't like silos. They didn't like their data swamp. They want to be able to manage and analyze diverse data in a performant manner, and the lakehouse can help with all of that.
Data Mesh Definition
Fern Halper:
So that's the short review of what we went over last time in terms of adoption. Now let's switch over to data mesh. You're going to see how these things intertwine, but I think most of you have heard the term data mesh. It's been gaining interest in the market. The mesh concept was introduced by a woman named Zhamak Dehghani in 2019, [and] she said something important about it, which is that it's not architecture, it's a sociotechnical paradigm. Read uses it as a philosophy, which I think is a good word also, and it recognizes the interaction between people and the technical architecture and solutions in complex organizations.
The Pillars of Data Mesh
Fern Halper:
She said that there were 4 pillars to this idea of the data mesh. And this comes from a pre-release copy of her book on the topic. The first is domain-oriented ownership. So the idea here is that business domains own their data, so ownership is decentralized. The idea is that this should scale out data sharing and the domains share their data across organizational boundaries. So, for instance, when we think of a domain, that domain might not be a business unit, it might be a supply chain domain, or [a] domain associated with products. So the idea, though, is that these domains own their data and that they can share this data, and this data is viewed as a product.
Data as a product is the second pillar here. So the idea is that data is accessible to those who need it. The data is viewed as a product, which means that customers of that product should be satisfied with it, and the company should be organized to support the product view of data. So the business domains themselves are responsible for things like data quality and understandability and interoperability of of these data products. And the idea is that data quality and understandability, they're going to improve because the domains are offering up this data as a product. And, they want to make sure it's interoperable, and the other domains are satisfied with it.
The third pillar is self-service. So here the idea is that a self-service platform can be used to empower teams. So multiple personas can make use of this self-service platform. The idea is that this will lower costs and enable the development of data products like dashboards or even specific products. So not just data sets, but developing products with data and developing data applications. And then the fourth is federated governance, and so in the data mesh approach, governance is based on a federated model with team members from different domains as part of the effort. And so the idea is that this data mesh model is going to balance the autonomy of the domains, but it's going to make sure that compliance, interoperability, and security of the mesh holds, so the domains are responsible for the governance. But what we've seen happen is oftentimes, there's a hub and spoke model where there may be a small centralized team that works, with the domains in this.
So these 4 pillars are supposed to help organizations overcome the complexity of the data environment, get to self-service, get to AI. Likewise, that's what the Data Lakehouse is helping. Organizations do, too. I will say that CDOs the TDWI interviewed say that they support a number of the data mesh principles, and they're already practicing them [as much] as possible. So, for example, they believe in coordinated and federated governance. They like the idea of data as a product, and many of them are moving to a self-service model. And so you could see how the Data Lakehouse could support the data mesh philosophy, being able to do self-service on the lakehouse, different domains can own their data as part of that lakehouse read, we'll talk about how that could work. And that will help to move the needle forward.
Data Mesh is Not Widely Adopted…Yet!
Fern Halper:
What we see at TDWI is that the data mesh isn't widely adopted yet. And I would say, this is data that comes from a 2022 survey where we asked respondents if they were utilizing the paradigm, and you could see that we put the whole definition there about the paradigm. And you can see that 15% said yes, but the majority said, no, but many of them like the data mesh principles. So 30% are saying, no, but we support the data mesh principles. So we have 30 and 15, so close to 50% are saying that they're either using it or they're using the principles of it. Others said that they plan to move to the data mesh paradigm, which was about 20%. And the rest about 38% said no, or they don't know.
So what we have is people who like the idea of it, and they're planning to use it. And, again, they like the idea of data products. We're hearing a lot about that from our audience at TDWI, and a lot of people talking about how they're building these data products. They like the idea of federated governance. A lot more of them are putting this hub-and-spoke model into place. They like the idea of self-service, that's a top priority that we see with organizations. We've seen them for the past few years. But I will say there's no real difference between those who are using a data lakehouse and their view that the mesh is a priority.
More than Half of Respondents to a Recent TDWI Survey Agree that Data Mesh is the Best Option for Data Management
Fern Halper:
So, they may be using the Data Lakehouse, and so, and still think that the mesh is a priority or not, because they like the principles [of] it. And then we asked that in a different way, too. And this is from 2023. So we asked respondents whether the data mesh is the best data management approach a weird question. But that's the way we asked it. And you can see here that 57% agree with this and another 31% are on the fence. So only 10% didn't think that the principles of the mesh were a good idea. And as they said, CDOs and others are telling us that they like these principles, and they're implementing some of these pillars. So even if they're not implementing the whole thing, they're implementing pieces of it. And, what does the Data Lakehouse have to do with this? Rita's going to talk more about that. But with platforms like them, you can combine data sources. Using a semantic layer, you can create multiple data products in a data mesh. Different groups can create their workspaces, So you have a solid data foundation while you support the pillars and the philosophy of a data mesh in terms of products, self-service, and so on.
Challenges with Data Mesh
Fern Halper:
Just to close this out, I wanted to share some comments that we got from organizations thinking about a data mesh and the challenges of the data mesh. And this is from 2023. And I think that there was a lot of confusion out there, back in 2023, because people were saying that they didn't understand it, that they appreciated the pillars, but they were concerned about things like change management. And they were concerned about the cost, and they were concerned about decentralized data, and the fact that they couldn't have governance. On the flip side, organizations who are using data mesh say that they've become more data literate, and they're able to implement more self-service. It may take a little while to get there, but they're actually––if the business, the domains are using their data then, and are responsible for the data, that means that they're going to become more data-aware, and then they're more data literate, and they want to do more self-service. And so, all of those things that were a challenge before become somewhat easier with data mesh.
Poll Question
Fern Halper:
So Read, does that drive with––oh, first I want to ask a poll question. Let me do that, and then we'll find out if it jibes with what Read is seeing. So our poll question is, what pillars of data mesh are most important to your organization? So I've been saying that organizations may not be implementing every pillar: domain-oriented, domain ownership of data-as-a-product, federated data governance, or self-service, but there may be one or more that they're most interested in. So, curious to see what pillars are the data mesh are most important to your organization, so you can select all that apply here, and I'll give you a few seconds to do that. As I said, we're hearing a lot about data-as-a-product, obviously, self-service. So it'll be interesting to see what people are saying….we're getting…coming in…coming in…[we’ll] get to a certain level here, and then I'll show you the results…or maybe everyone's picking all four pillars of the data mesh. We'll see what they say…let me see what they're saying here, I think we have enough to get an idea…so, interesting.
Read Maloney:
A lot of balance.
Fern Halper:
Yeah, and 13% say, we don't ascribe to data mesh…so looking like, well, obviously, a lot of self-service, but a lot of balance. So Read, does this jive with what you're seeing?
Read Maloney:
It does, and Fern, your data is a lot from the 2022 survey you did, we did one in 2023, quite recently, and we see much higher adoption in general of data mesh and data mesh concepts, or, as we were talking about, the data mesh philosophy within an organization. And it just shows––obviously, GenAI jumped in and it was a massive topic, and we're going to talk about AI also today––but data mesh was a really hot topic within organizations.
Data Analytics Nirvana
Read Maloney:
And one of the things that we think is the driver here, is like, just what are you trying to achieve in the organization? So it's like, data mesh as a philosophy––the concept doesn't only mean anything. Why is it popular? Why are so many people adopting it? Because it drives business value, or at least is driving perceived business value. And then, as companies realize it, they talk about it, and then the other companies start to adopt it, and you have that adoption bell curve, as you go through. So if you think about the adoption of data mesh, are we in the early majority or late majority, like we're actually, I would say, deep the in the early majority based on the data we have, and it's becoming more of a standard. The reason is that companies want to drive more data adoption; they want every organization they have [to use] data.
I think one of the interesting things is, in my career, I've been both on the engineering side, doing a lot of statistical analysis, using tools that, I would say, people don't use anymore and are now more associated with data science. And now [being] also on the business side, and being like, how do we bring all this data, even? We drink our own champagne. I have my own Dremio Data Lakehouse from a marketing perspective. We need to bridge all of the different sources together and create the views we need to go, and our business wants that because they want us to move fast. And I have way more questions to answer all the time than I can answer. So I need to lower the mean time to insight. And we consistently hear this from customers, like, we want to drive more data adoption, we want to lower the mean time to insight. And the problem is, as you do that, two things happen. The business is just exploding in terms of usage, that's a cost problem, potentially, and I think with some other technologies that they've seen on the market, it's also a scale problem. So cost or scale, and the lakehouse can help with that and some things specifically Dremio does, that help as well. And then you also have this governance problem that comes up, and I think you saw it in your challenges, Fern.
All we want is one very large central data engineering team with one source of the truth. That is a view to have, but it's not the view we've seen from customers who can start moving quickly and expand data within the organization. We've seen that hub and spoke model that you talked about earlier quite a bit within our customers, I don't think we've seen full distributed data ownership yet from customers, although you'll see in the survey, some people claim that they're there. But I haven't quite seen that yet. I don't know, have you seen that, Fern? Have you seen customers say, the central team does not have any ownership of data assets, or data products depending on what terms they're using? I haven't seen it yet.
Fern Halper:
I haven’t seen that yet. And there was a question even in the Q&A box about that. I haven't seen it yet, in terms of every domain owned, that hasn't happened. I mean, I think it will be, there may be some evolution there, but I haven't seen it yet.
Read Maloney:
Yeah, I see a hybrid, like we see the central team having ownership and domains, having ownership. Often, with a set of central governance controls that are applied across all. Our platform can help support that, but there are other tools as well, like Previcera, for example, that can be used to enforce policies across, and so I think this term of like, hey, you're just going to set your governance, isn't really what federated governance means, and we'll cover that a little bit more.
Obstacle: Complex Data Pipelines
Read Maloney:
One of the things that we continue to see, though, and this relates to the challenges of, hey, I want more adoption, and I want a lower mean time to insight, and I want costs to be lower, but what happens is––still, traditionally, I got all these sources. I gotta write all these ETL jobs to get into a lake. I then don't want to make that performant for the end users. I'm going to go put it in like a star Snowflake schema, and I'm going to go make another copy of that data, and I'm going to then have a longer ETL chain to manage. And then on top of that, we're going to get extraction cubes and other types of computation for performance.
And so to do any of this as any client, any customer, or consumer of the data, I have to keep going back to the group who can write those, and the more those require specialized skill, that hits the central team, or it's going to hit at least some specialized data team, even if the department or line of business is big enough to have a data team. And so this continually stands in the way of self-service, and the first part of that is, is a lakehouse. So Lakehouses in general, this is outside of Dremio specifically, and we'll talk about some things that we do to help from a self-service and data mesh philosophy construct, but in the lakehouse, specifically, what you see is you start to shift the data consumer closer to the source, which we think about as shifting left. And so what has happened in this––well, by removing the warehouse now, we've eliminated an entire staff ETL chain. We've eliminated a copy. We've eliminated management. We've eliminated another point where you have to go back to the engineering team to be able to create the view that you need to be able to work on the data. And then again, if you think about data products, now, I have to have a product that sits in the lake, and then I have to manage that product again into a different data model that sits in the warehouse, and that's complexity now. And complexity overall is going to kill self-service, that's going to mean everything goes back to a specialized resource we don't want, and it's going to be more [costly.] So all of those things that we think about as data analytics nirvana start to break down. So this is a good step. And I think that we'll see, though, there's still a bunch to manage in them. So it's better, but it's not sufficient.
Lakehouses are Great, but They Don’t Enable Self-Service Analytics
Read Maloney:
And so when we look at data mesh in general, what are organizations going to try to achieve? Trying to achieve again more data adoption and lower mean time to insight, is going to come from enabling the business to do analytics or self-service, and one of the pillars is a self-service platform. But if you look at data maps across the pillars, distributed data ownership, you look at federated computational governance, etc––that's all based on shifting left, getting the consumer closer to the source of the data. And that's really where we believe we come in from a platform perspective.
Shift Your Data Analytics Left
Read Maloney:
So we think it requires three core pieces of innovation to do this. You need an intelligent query engine, and I'm going to talk about the importance of that, and how that works. Because, if not, you're always back to the central engineering team, whether it's the engineering team in your business department, the central team, or both. Sometimes you gotta wait in a queue just to figure out you have to wait in another queue. The only reason I know that is my teams have done that before. In the past, we've had to wait, and then you wait again, and by the time you need that insight, it's too late, you're already on to trying to make the next program––in my case, the marketing program––work or not, and you're going for imperfect data instead of better data.
You need an intuitive self-service experience. For example, I'm going to have analysts on the team––are they going to write Python? No. Are they going to write SQL? Maybe. Are they going to be able to create a view if they don't write SQL? Well, I need an experience to do that. GenAI is helping here, and we put that into our product as well. And we'll talk about some of the elements of like, hey, I want to be able to create this view, and now I want to query the view, and I want it to run fast––how does that happen in a self-service environment? That's going to be paired with a query, and I'm sure we're going to talk about those two together in the next slide, and then we're going to talk about the next-gen DataOps capabilities. And this is related to, how are these data products––how am I going to know that I'm working off clean data? When I get into self-service, I have this whole problem, which is, that I'm working on these data sets, and if they're not managed or supported, then I'm working on data that's not being updated that I'm expecting to be updated, and I don't have that information. And now I've created a view that doesn't update, but it updates in some other element. So maybe I'm getting data in from Oracle or a different product analytics set like Eep, then I might be getting data from GA, Google Analytics, and then I might be getting data from my CRM, and now the CRM stuff’s not updating cause that's not managed, and I'm running the reports, I'm in trouble. I have to know. So that's that's part of the next end.
Shifting Left Shortens and Simplifies ETL Pipelines
Read Maloney:
And so what we're looking to do is, again, shift as far left as we can. So here's an example––I think the formatting as we move this one over got a little bit changed––but you can see we have two sources coming in. We have voter data, and we have voter survey data. That's an actual physical copy coming in, that's the purple, whereas green is a view, that's a logical view. And in general, the business should be just working with views. You don't want them to have to create a copy every time they want a view. They should be able to just work with a view logically, so you're just managing the actual source data coming in. That's the first thing. So instead of ETL, you're moving the ‘E’ out. But we're just loading from the source. And then I can create a view that's pulling all these items together, so in this case, I'm going to pull the voter data and the voter survey data into an enriched voter data view. And then I'm going to have, maybe I have a group that needs to work out of this in Ohio, there's an Ohio view, and there's an Illinois view, and then I might, as an end user, have multiple pivots of those views. So even another layer of views.
And so the issue that can happen as you do that, because unless I'm doing an extract into a BI tool for performance, you don't want––now, I don't have a common semantic layer definition across the organization. I also then have to manually update that. It's not updating in real-time, every time there's a change to the data. So now I have more management complexity, but I still need to manage performance. And so one of the things Dremio does is we will recommend the materialization––so you see these lightning bolts, that's [what we] call a reflection, so it's a materialization of those views. But our query engine is intelligent enough that if the materialization exists, we will rewrite the query the business is using on the view. So let's say, you're just querying Ohio voters. I'm writing that query to Ohio voters––I’m the business user––okay, great, it would usually have to go through the sequence of SQL to SQL to SQL, and that could take a long time, and it's not necessarily price performance. In this case, our query planner's smart enough to know there's a materialization that exists, and it will just rewrite the SQL itself. That's what we mean by an intelligent query engine––it will rewrite the sequel at runtime to take advantage of the materialization to manage performance. And so now, the engineers, we're alerting them and saying, hey, guys these are the views you're going to want to materialize. So they only do the ones that they need to do, and the query engines are rewriting it in real time. And we fundamentally believe you cannot do self-service without that––this is a self-service platform piece of it, which is, now, as a data engineer, I'm bringing in and managing the source data on an EL, and I'm thinking about this data file.
And then the vision is, from there, everything's logical except for the recommended materializations that are used, and then the intelligent query engine has been reading off those materializations, and you're maintaining a great performance and price-performance balance. That’s what's going on, and so to be able to do that, all these views [need] to be easy. And so that's something in Dremio [where] we make it simple to connect whole different data sources, apply governance in terms of row and column level access controls into the different groups that you're doing by department. You can have engines by department, they can go out and just run the views that they need, back them up, they can create them in a no-code environment, they can even do text sequels, as they need to in the experience, and they don't have to think about, hey, who do I need to talk to make this work better or return [from]? We're going to return that to the engineering team so that they can move quickly, provide the materializations that are required, and then let the query engine decide on a rewriting of the SQL on the fly.
Data Mesh Adoption
And so one of the things we talked about, Fern, is the difference between our data. Where you have [from] 2022, and we have from 2023, our data says we have 84%; you had 13% on the survey [saying], hey, we're not doing anything with the concepts. And we had 50% come back and say, hey, we're fully implemented in data mesh, with another 34% saying partially implemented. So that shows a massive adoption, but you're going to see on my next slide that 97% plan to continue implementing data mesh. So there's something a little bit up with, how can I have 97% saying, we're going to keep implementing when 50% say they're already fully implemented? So what I think, what we interpret that to mean is that 50% are working on all the pillars. Like, there's an element of effort, sort of like when you polled the audience and we saw a split across what was happening in the different pillars. [Our view is] that there's there's a data products effort, there's a self-service platform effort, there's a distributed data governance effort, there's a domain ownership effort. And we think if there's an effort there, we probably saw that reflected in the data ‘fully implemented.’ But you can see how much this moved. And this is pretty darn close––our audience, 13%, said they haven't done anything with data mesh, we don't have plans. We have 16% saying that. So that's how much the market's moved in the year. I think your data said 45% last year [said] that we're in the fully to partially implemented phase that jumped to this 80-ish––we don't––this is a 500-person sample and today is about a 100-person sample––so, you start adding all that together, it's in that 80% range.
Fern Halper:
I do think that they're working on pieces of it like, the data product thing, like what you're saying, data products, [are] definitely big, definitely a hot trend in thinking about data products, Distributed governance. I think the domain ownership––I don't know where that is, I haven't seen if that is something that organizations are completely working on, because it just seems, at least, people we talk to, and they're still trying to figure [it] out. A lot of them, they either want to have some logical or physical architecture to have some unified view that, I'm not sure if IT is still controlling, or if the business is controlling, you know what I'm saying?
Read Maloney:
So, I mean, we talk to a lot [of people] because we provide this unified lakehouse platform for self-service analytics to the market. We end up talking to a lot of central teams. Initially, we had talked to a line of business teams as well, but again, I think it's a hybrid. It's like they're trying––their leadership is trying to empower the business. And it's a journey, absolutely a phased approach. Like, I don't see anyone that's like, we're just setting rules and the business is doing everything else. I haven't seen that, like we talked about at the beginning. So I think this is ‘fully implemented’ means––we have some implementation across the different pillars of data mesh. That's how I read that. Or we have some working model for self-service analytics in the organization.
Fern Halper:
Yeah, I agree.
Read Maloney:
There is a question, I'm going to jump into one real quick. Just hey, the structured format in a warehouse versus a lakehouse. When you say like, hey, I'm talking about EL, are you doing a load, is there a transform? Yes, in our world, we see that going to Iceberg. So you would take the raw data that you have, and it's still a raw load. But it's a raw load transformed into Iceberg. So there, there is technically a transformation in there, from one format to another, but it's still a raw load, and we think about the load landing in a format like Iceberg, because then you know it landed like you still [can] say it was written at this time, or guaranteed that this was written. And so the table format gives you that ability like it would in a warehouse.
Number of Data Sources for Analytics
Read Maloney:
The next thing is the number of sources for analytics. So this was a fun one, in which 50% of the 500 organizations we looked at have over 21 data sources within their organization that they're managing. And so there's a lot of push, people do want to consolidate. But the reason why they want to consolidate is they want to unify access to data for the business. And why do they want to do that? They want more data adoption. They want to lower mean time to insight to drive value for the organization. And so we see a lot of customers, they want to consolidate, but they know that's a journey. And some of them, Fern, like we've been talking, I think you said some of your customers want to keep data where it is. Is that true?
Fern Halper:
Yeah, yeah. I mean we see that the median number of data sources is about 15. So close to what you're saying, although a lot of companies have thousands of data sources, and that depends on how you define a data source.
Read Maloney:
Yeah, we didn't define it for them in the survey, but, we saw that, too. 10% of over a hundred. And so the way to do that is from more of a fabric concept, that Dremio supports both on-prem and in the cloud. And so you can connect to these different sources and start to create that unified view, that unified environment, unified semantically or across the whole organization, that's great. But we know that in some cases you're still paying the licensing fees, you're still working with that group, and you do want to migrate that into your lakehouse. And so customers have different views of what they want to leave in place, and what they don't, and what the top priorities to move are as they think about price performance, trade-offs, and delivering that to the business. So there is a lot out there and this is a key element of data match, which is just the unification of data.
Data Mesh Initiative Leaders
Read Maloney:
The next one we talked about is who's driving the data mesh initiative. And so I found this fascinating, which is, if you look down here, there are 32% [are] data teams within [the] line of business, and another almost 9% were from the actual departmental leaders, such as a CMO or a CFO or a CHRO, etcetera, and if you add those up, 41% [is] coming from the line of business, whereas from the central team is 36%, and that, on the whole, doesn't surprise me, because, the central team needs to have the mindset [of], we want to empower the business if they're going to take the lead. So there's a chunk of those groups out there that are like we are going to help push the business forward and drive transformational and digital change in the business. And then there's a group where that's not happening, and then the business takes the lead and says, no, no, we need this to be able to go. We're not moving fast enough. And then in other cases, it's such a priority, that it's coming straight from the Digital Transformation Office or the CEO.
Fern Halper:
I thought that chart was really interesting.
Read Maloney:
Does this align with what you've seen, Fern?
Fern Halper:
We still see that. I agree with what you're saying, we've seen that organizations, if they don't think IT is moving––the central group, whatever you want to call it––isn't moving fast enough, then they're going to do what they want to do to drive the business forward. But we still see a large percentage of those leading any type of effort coming from some central organization, it's not the business. But it was only about 50 or 60%, so it could jive if you have 32%.
Read Maloney:
An observation I'll make is that if there's a CDO or a CDAO within an organization, it's almost always their organization driving it. And in cases where that's not the organizational structure, we're more likely to see the business driving it.
Fern Halper:
I mean, that's their job to drive value, so it makes sense. We just ask our questions [differently,] but I agree with what you're saying here.
Why Data Mesh?
Read Maloney:
And then, why data mesh? So the number one reason for why data mesh, is data quality. So that was that makes sense to me in terms of the fact that data quality is so important. But it also is something I would have expected to see more of, honestly, from, agility, time to insight, and improved data access, improved decision making––they're all in there, and they're all pretty high up. But the actual top two are governance and quality. My interpretation of that is on what was the version of ShadowIT when I was working more in the cloud computing environment, which was the business was just outdoing stuff to try to move fast and meet their needs, and or maybe going outside the construct of what they were supposed to be doing. And so part of how I was reading this is, look, they're doing a bunch of manual things, they're doing a ton of extracts, I mean, extracts are common. And in doing so, that complicates governance. It also complicates quality, and it complicates a common semantic definition which has an impact on quality because you might be using the same number, but you're defining it in two different ways across two different teams.
And so that was how I viewed the response because I was expecting to see a little bit more agility, [and] time to insight when we looked at this.
Fern Halper:
I think data quality always comes up in every single survey. It's a top challenge, it's a top reason. People are thinking about data quality, and as they're moving to AI, [as] we're going to talk about next, they're very concerned about the quality of their data, not only for garbage-in and garbage-out but also, they're collecting new data types, that they're putting into their data lakehouse. And they want to make sure that the quality of that data is there, and data mesh could help with some of that, and see it better too.
Read Maloney:
So, Fern, Jay just asked a question about the survey, so I just want to jump in real quick. Number one is the n is 500, Dremio did not administer the survey. That would be a bias towards our customers or a group––it is a broad market evaluation of both practitioners and leaders. We have segments from 10,000 plus, 5,000 to 10,000 employees, and 5,000 and below. More of that is covered in the direct report––State of the Lakehouse 2024 that you can look at.
To Further Simplify Pipeline Management, We’ve Created Git-inspired Data Version Control
Read Maloney:
And the last slide on this topic, I'm going to try to move this quick. This is the last element of next-generation data operations. And so we have created a git-inspired data version control. The great part about this is that it’s going to relate to data products, and it's going to relate to data quality. And so I'm going to focus on the first branch, and the second branch here at the bottom is going to drop us into the data science discussion. So what ends up happening is you have your main branch of your data. So this is like bringing software development best practices to your data. And so you have that asset, and then, you're going to be adding new information to it. And so before you want to make that your production set, the business can work off of, you branch it. And this is just a pointer, it's not a copy. So you're branching the data, you're then adding the data into the data set. You're then running quality checks against that data. And you can do that through whatever you're using, from a customization or tooling perspective, things like Great Expectations or Monte Carlo, and then, once it passes the checks you merge it in. And so in that process, if there are any issues, you know exactly where they occur, and when you merge and you version the data. And so at any point, you can roll back to a previous version, and so this helps a lot in terms of managing data as a product, and then also, if something happens, troubleshooting back.
The other thing that we'll talk about, and this is going to relate to the AI conversation is, that I can create a branch for experimentation. So instead of having the data scientists go off and create a complete copy of the data, I can create a pointer back to the main branch. I can then add the different data that I want into that branch, and then I can do feature engineering on that branch. And if I end up developing features that I want to keep, I can put those back into the lakehouse, etcetera, and when I'm done, I can just drop the branch. And I can do all this without creating a whole other copy of the data. And so this is something that Dremio offers, and we refer to it as git-inspired data version control, because it's one of those elements of software best practices that's getting brought to data.
Data Lakehouses Help with AI
Fern Halper:
Interesting, someone just asked a question about data quality and data lakes. And, it's a bigger answer, but let me talk a little bit about how data lakehouses can help with AI, which was the next topic that we wanted to talk about. And the first, [which] we've talked about this already, is that they can help bring massive amounts of diverse data together for use in AI and eliminate silos. So, a lot of people when they're building more advanced models, they're looking to get an enriched data set that they can use to build meaningful features and to train the models, and the data lakehouse supports the diverse data and massive volumes of it, which is often needed for training. [Also], since the data lakehouse is unified, that makes pipelines easier to manage for diverse data and AI. Read, you were just talking about the pipeline, so I don't have to go into detail there, and I just mentioned that it can support compute-intensive workloads. And also many data lakehouses have marketplaces associated with them where users can buy and sell data and data products. And, these data products can run the gamut from things like datasets to notebooks, even to models that those organizations want to share, and they can also help with data enrichment. So it's not just the data that's in the data lakehouse, but the marketplace that's associated with the data lakehouse might have weather data, demographic data, industry-specific data, and all of that can be used in the models that you're building. And obviously, that can help with AI. These data lakehouses also can support different user types, we were talking about business users for some types of self-service, as well as data scientists.
The Data on Those Using Data Lakehouses
Fern Halper:
I have a lot of data in this chart, but what I did was I took a lot of the data that we had about the data lakehouse and AI because we run surveys all the time. And then I was trying to compare those who were using a data lakehouse and AI, with those who were using some other type of platform, so trying to compare them across what's more likely with those using the data lakehouse. And so you can see here, I'm saying that those using the data lakehouse are more likely to collect higher volumes of data. So I'm looking at where there are big gaps here, like 47% versus 34%, they're collecting hundreds of terabytes and petabytes of data. We talked a little bit about generative AI, but those using the data lakehouse are also more likely to be using large language models and other advanced tools on unstructured data. So we're seeing 40% versus 25% for large language models. We're seeing 45% versus 27% for image recognition, and 50% versus 26% ETL for unstructured data. So they're trying to make use of some sort of unstructured data versus those that may just be using data warehouses and data lakes. So they're more advanced in terms of what they're doing. They're more likely to say that they're using diverse data to support innovation, and they use marketplaces [too]. So I have these statistics there. And, by the way, in one of our research reports, we see that those organizations that make use of data marketplaces are more likely to monetize their data. So, it's just all like one big virtuous cycle in some ways. [If] you want to move forward, you need a good platform, you can use a platform like a data lakehouse, use the data marketplace, you're doing more innovative work, you're doing more advanced analytics, you're more likely to use cloud services for processing. You're more likely to say that you've derived insights that you would not have been able to alone with structured data––63% versus 51%, So as I say, at the bottom here, it's more advanced in their analytics and AI efforts, But still, early days, because this was those using the data lakehouse, it was a relatively small sample size to do the comparison with.
Other AI Maturity Indicators
Fern Halper:
But they have other maturity indicators, too––those using data lakehouses also have more data scientists. I said the data marketplaces thing––they're more likely to have a committed leader in place that's going to help move the organization forward, with analytics. They're more likely to have KPIs to measure success. Read, you were talking about mean time to insight, they're measuring what they're doing and using the right metrics there. They're focusing on analytics products. They're monetizing analytics. They're more likely to have an ML Ops team in place because they're putting more advanced analytics into production, and they need Ops teams to help them do that. And there are oftentimes larger companies, which is interesting, too, because they have the resources to do so.
There Are Still Challenges
Fern Halper:
Of course, there are still challenges, and these challenges are at the same level whether or not the organization is using a data lakehouse. So, for instance, it isn't as if using a lakehouse is going to automatically make you able to perform advanced analytics. Yes, the tools are available, but whether or not you're using a lakehouse, you could still experience issues in advanced analytics, like here I have one extracting entities from text data or labeling images. They still report issues with data governance, which is always one of the top challenges organizations face, and I think oftentimes, it's because they haven't thought through governance before implementing their new platforms or use cases or supporting new data types. It's really important to be proactive with data governance, because there are tools on lakehouse and other platforms, but the tools alone aren't going to make you successful.
And here we go again with data quality. I think this is interesting because the data lakehouse can help to standardize data, help make it more consistent, but what we see is that a lot of problems with data quality come from all of the organizational factors surrounding data quality, such as putting policies in place, and getting people to buy into data quality. I was just looking at a chart the other day that had––it wasn't about identifying data quality, it was all of these other organizational issues around data quality that become an issue. There are tools out there that can help identify data quality issues, at least in some structured data. I think organizations are going to have to think about what data quality looks like in unstructured data. How am I going to ensure data quality if I'm doing vector embeddings, I have encodings, and generative AI? How do I know that I have the word bank, which has multiple meanings and is encoded differently and correctly, as part of my generative AI model? So, there are all sorts of issues around data quality. So do those results jive with what you're seeing, Read, on your end?
Read Maloney:
I mean, yeah, absolutely. We did an event yesterday with our customer, Maersk and we got a lot of questions around, with LLMs, around hallucinations. And you can imagine how important data quality comes in those cases which is even with, we'll say, high data quality and even limiting the scope of what that LLM knows to prevent more, you could still get those. So I think the bar, as AI continues to advance beyond machine learning, data quality becomes an even bigger focal point, and knowing what the sources [are] that you're training the generative AI off of, versus just using the full open model. That's why we're seeing, I think, more customized LLMs from organizations that we're talking to. And I think it's something that does stem from quality, you don't know what's in the background, there's just so much in there. You want to have a better understanding of what's been used for training, just like you would from a machine learning perspective.
AI on the Data Lakehouse
Read Maloney:
So a couple of things that we have on data from our survey here––again, I'm going to be cognizant of time, because we only have 7 minutes, and we have a lot of questions. I'm doing my best to answer them at the same time as they come in, we will try to get to these as much as possible. Because data lakes were used extensively, because they could combine structured, unstructured, and semi-structured data together, data scientists jumped at that. And AI has been the core use case of the data lake, and what does the lakehouse bring to it? Well, it's brought more analytics capabilities and performance, especially around the structured portion of that with table formats such as Apache Iceberg. And so it's not surprising to us that we see 81% using a lakehouse for AI, and in some ways, I'm surprised that we're seeing 19% saying they're using a lakehouse. And they're not using it for AI like they would be using it just straight as a traditional warehouse. I think that's in line with your 80/20 rule of adoption, sometimes there's always going to be a group, and it could be on an interpretation thing. But I'm not surprised to see this number be high. If anything, I expected it to be, maybe even 90 plus, in terms of what's happening, because you need that flexible store, you need a place to start unifying your data for the data scientists. So that just aligns with what I'd expect, and then––I think we skipped one there. Oh, nope.
AI Models in Production
Read Maloney:
So then, [onto] the next group here, we're looking at how many models are in production. And so in my past, in my past life, my past career, I worked at h2o.ai, which is an enterprise platform for building and managing models much more quickly, and we saw a lot of models end up in the garbage can, because, again, just the engineers would be up building something, scientists were, but they didn't get the business requirements first. And that was the number one concept, they weren't building for the business. And so we've seen that change now, I mean, if you're starting to look at the number of models in production on average, you saw about half were at 30 models now, in production, that they're managing. And so that's not just consistent training and retraining and watching out for drift and managing those models from an MLOps type of perspective, that's then, okay, well, what's next? And I think this is still very early days if you think about AI eating software from a conceptual perspective, if you think about generative AI just fundamentally changing business productivity, this is early, and I expect we'll see next year, this average would be well over a hundred. Like, I think there's a massive amount of move from hype and ‘what do we do’ to actual adoption and team delivering value. And so that's what we've seen. Fern, does that align with what you've heard from customers?
Fern Halper:
We don't see as many models in production, but the numbers are increasing. It went from an average of 5 a couple of years ago, and now it's up to about closer to 20, 15 to 20. So, yeah.
Read Maloney:
I know there are some enterprises out there with well over a thousand models in production right now, really core critical elements there. And that's not all generative AI, a lot of that are those are machine learning models.
Fern Halper:
And even to make small decisions, it's just embedded in their processes.
Will AI Be a Force for Good?
Read Maloney:
Exactly. We also asked a couple of questions that were just interesting around AI and maybe less on the technical side. But, like, will AI be a force for good? Well, at least within our technology group, most of the people were talking to their data practitioners or data leaders. This is not a main street one, but 89% think AI will be beneficial. So there's certainly a lot of focus that people are like, this is going to work for us. Fern, [do] you think you're hearing the same thing from your group?
Fern Halper:
Although I guess I think about all the legislation, all the political concerns about AI and the legislation. The EU just passed the AI Act the other day, so––
AI and National Security
Read Maloney:
––And this is the next piece of that, which is while people think it'll be beneficial, 84% do think it's a national security priority. So it's the regulation, the governance, responsible AI [is a] trend, AI Governance is critical, and you can see there's going to be much more regulation. And, I think in general, people in the data analytics space believe there should be regulation because they want it to be a force for good. They don't want it to be something that's out there, for, nefarious reasons, or things that come back and we're like uh oh, that wasn't a good idea. Things like, where does a human need to be in the loop? Concerns around AI governing AI, how does that work? And it's being done today. So, where do those lines go? And that really is what I think makes AI a national security priority.
Fern Halper:
We're definitely seeing more organizations interested in AI ethics. And they're going to have to be, moving forward, responsible [with] AI. All of that.
Summary
Read Maloney:
Got it. So just a real quick summary from our side, if you want to improve data adoption and reduce your MTTI while reducing your costs, we think you have to have these 3 innovations. These are 3 innovations that Dremio specifically has, that are not available by others in the market. And so that's why we think we're seeing a lot of adoption and growth from some of the largest enterprises in the world now.
Download the Full Report
Read Maloney:
We do have the full report––give me a moment. If you want to grab that, that's from a QR code perspective, you can also just search 2024 State of the Data Lakehouse, Dremio, you'll find that on Google.
Get Started With Dremio for Free
Read Maloney:
You can get started for free. We have also a software version. So if you want to just get going, playing on your laptop, you can just use a Docker container and get going, and we support Kubernetes. And then obviously, we have a cloud environment as well. Those are all free. There are no time limits. And so you can play around and experience this, everything I've talked about today, for yourself. It's all there.
And then, I know we're pretty hot on time coming in here, Fern.
Q&A
Fern Halper:
Andrew, do we have time for one question, do you think?
Andrew Miller:
Yeah like, why don't we squeeze one in there, maybe 2, if we have the time? So really do appreciate that conversation. Those presentations were fantastic, and I'll jump right in here. Read, this question will be for you––how does Dremio support the creation and management of Beta products?
Read Maloney:
Got it. So we were talking about data products earlier in the data mesh section as one of the pillars. And if you remember, on one of the slides I talked about git-inspired data version control, which is something that Dremio offers. And so what we enable the customers to do, is take their data sources, and as they're loading them up, version them. And so they can say, look, this is version one. And every time we're going to be adding new data from the sources we're going to ensure that it's high quality because we're going to branch it, we're going to check for quality. By the way, AI is being used pretty often for quality checks, and anomaly detection type of models. And then it goes and bridges back in, and now I have the source of the data within the lakehouse construct that's available for the business. That is a way to manage that as a product, as a data product to the business. And so it makes it a lot easier to do that. And then the other constructs around the governance of that product is that we will re-enable you to set between the different groups you have, in terms of your marketing, finance, etcetera, different controls, and then you can do that by person. And so you can do row and column level controls on your data for those people, and so [the] data product can then be managed, and from a governance perspective. And those 2 things work together so that when you're enabling self-service, you're doing so in a governed manner. And if performance keeps working, it's easy to build views, you then limit the need for the business to try to go out and think they're going to do extracts, which then again leads to its own governance and security problems. Where's that data? Is it flying around? It's in the spreadsheet, or it's over here. And so by giving the users a better experience, it actually helps back into quality and governance for the overall team. Hope that answered the question.
Andrew Miller:
Excellent there, I'm going to squeeze one last question in. You touched on governance there. But Read, how does Dremio support governance in a distributed environment, please?
Read Maloney:
Yeah, I mean, that's what I was just alluding to because you can unify across sources. So within Dremio, you can add RDBMSes, and enterprise data warehouses like Oracle, Teradata, etcetera, along with your lake, and then you can set access controls across those different sources. And so the other item is, we also have integrations with companies like Previcera, so that if there are policies that then need to be enforced across the organization, those come in, and you're enforcing those. So the central team can then provide essentially a platform for governance that allows the distributed groups to go and run fast, but doing so in a governed environment. And that's the most common model we've seen from our customers is, instead of saying, hey, marketing team, go govern yourself, if the data comes over with a set of policies, in terms of like, who can see what from the start, and then you can request access in, but then, if I'm bringing our data into the source, I would own that. And then the team's central team would need to say, yes, we agree with how that's being managed, and that's a little bit more of the hub and spoke that we see in our customers.
Closing
Andrew Miller:
Okay, fantastic. Well, we did run out of time a few minutes ago, but thank you both for staying on a little longer for everyone here and please allow me to thank our speakers. We did hear from Fern Halper, with TDWI, and Read Maloney, with Dremio. I'd also like to thank Dreamio again for sponsoring today's webinar. And lastly, from all of us here, let me say, thank you so much for attending. This does conclude today's event.