NEW YORK CITY + VIRTUAL
7,000+
Global Data Experts
60
Breakout Sessions
3,000+
Companies
That's a wrap!
Thank you all who attended Subsurface LIVE 2024
In 2024 we explored the latest open source innovations in the open data lakehouse ecosystem. We showcased real-world applications, explored the benefits of Apache Iceberg, and gained insights into the exciting developments in AI. We heard from companies like Apple, AWS, Blue Cross, and Vanguard about their experiences architecting and building modern cloud data lakes.
Sign up today to be the first to receive updates about Subsurface 2025
featured speakers
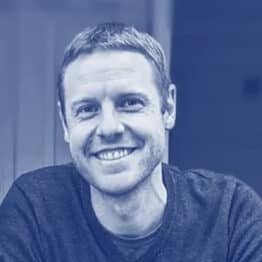
Ryan Blue
Co-founder and CEO
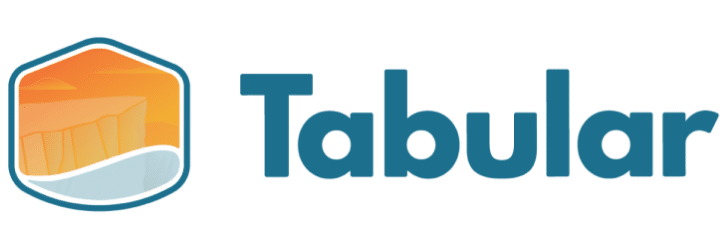
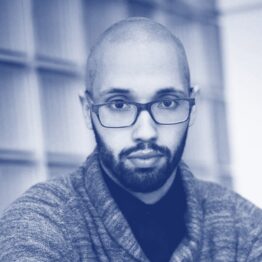
Chad Sanderson
CEO & Co-Founder
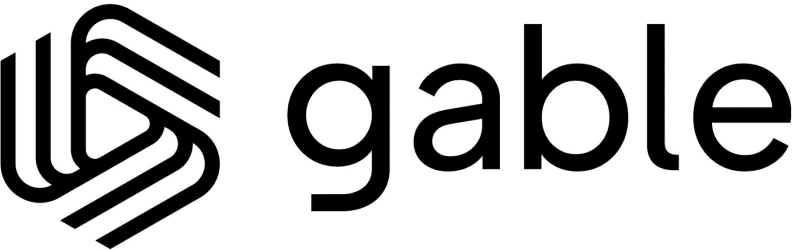
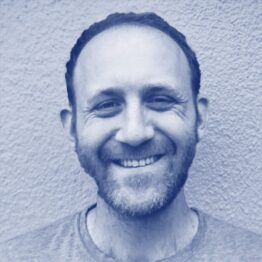
Russell Spitzer
Software Engineer at Apple
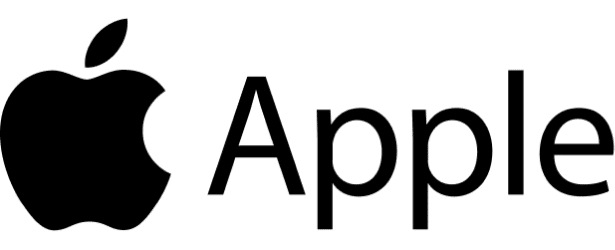
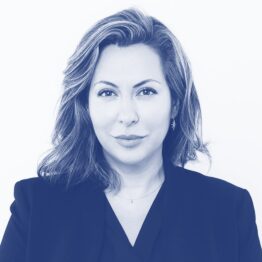
Zhamak Dehghani
CEO and Founder
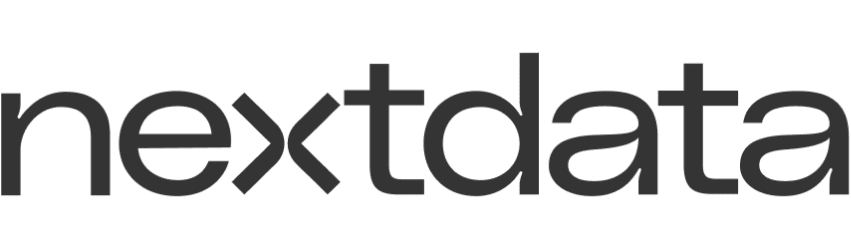
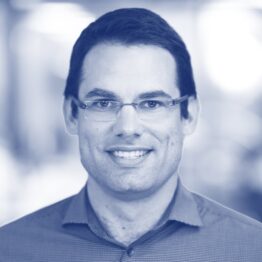
Tomer Shiran
Founder and CPO at Dremio
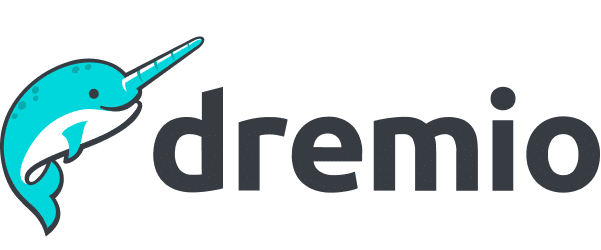
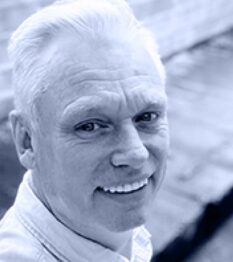
Mark Sear
Director of AI, Data and Integration
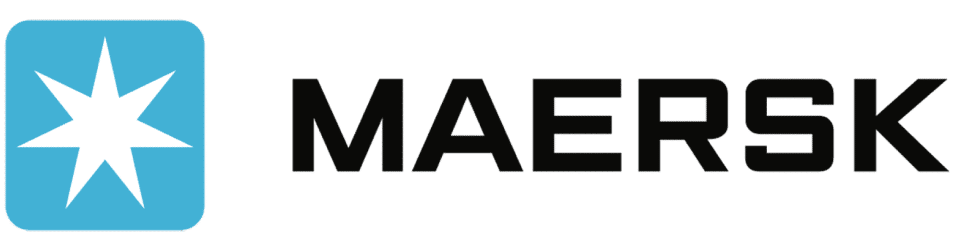
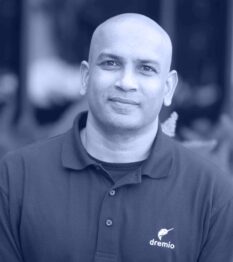
Sendur Sellakumar
CEO at Dremio
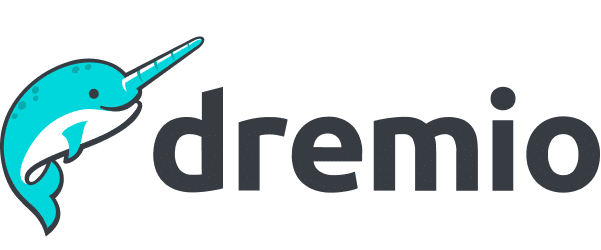
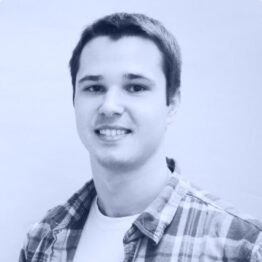
Anton Okolnychyi
Software Engineer, Data Infrastructure at Apple
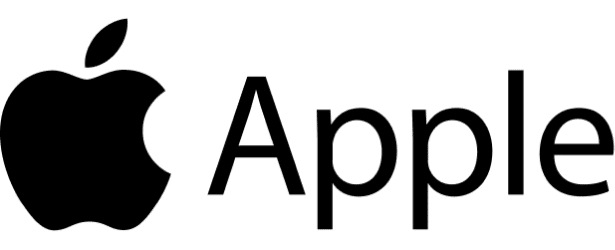
Subsurface Sponsors
Platinum Sponsors

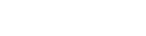
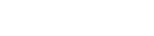
gold Sponsors

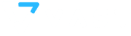
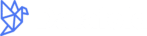
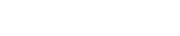
silver Sponsors
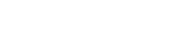
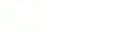
virtual Sponsors
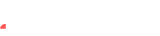


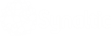